
Personal lines solutions
Optimise your portfolio performance
properties.trackTitle
properties.trackSubtitle
Insurance Consulting is Munich Re’s in-house consulting unit. From underwriting, pricing, analytics to product development, portfolio management and claims: we provide a comprehensive suite of consulting services along the entire insurance value chain.
More than 60 insurance experts around the globe combine data, technology and in-depth domain knowledge to drive your performance and create real business impact. Partner with us: we are your trusted advisor – and share the risk!
Enhance your insurance pricing and underwriting

Your challenge as a Property & Casualty insurer is to be strong in product development, pricing and underwriting in order to stay competitive. The digitalization process has caused a deluge of structured and unstructured data.
Let’s use them to enhance your pricing and underwriting process. Benefit from our expertise: we continuously improve our set of tools, procedures and techniques to stay ahead of the curve.
Benefit from pricing models
Get the full picture of our consulting services
Discover our success stories around pricing
Create a leading digital insurance platform
Improve growth on new business
Protect the market share
Bring risk pricing to the next level

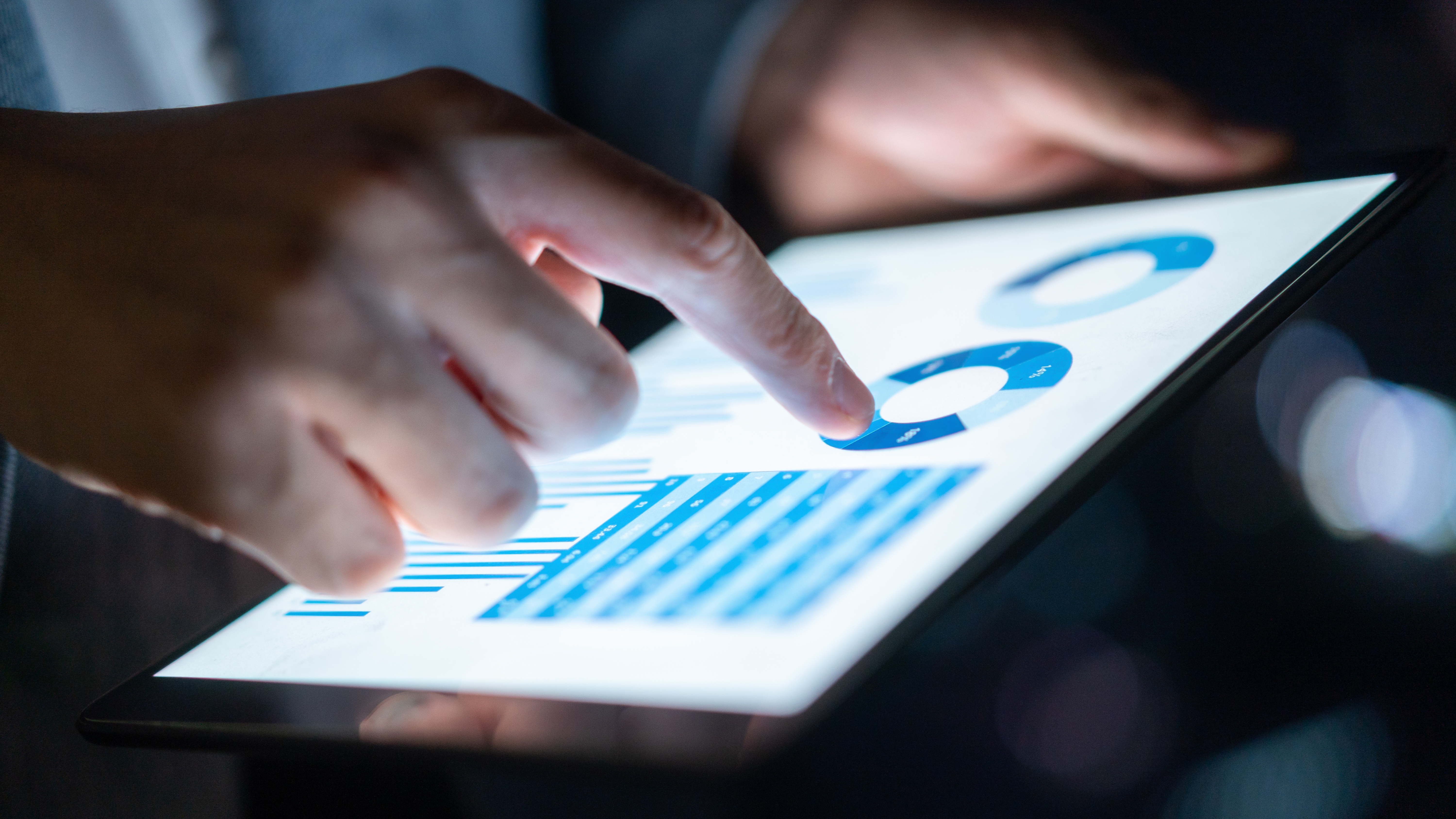
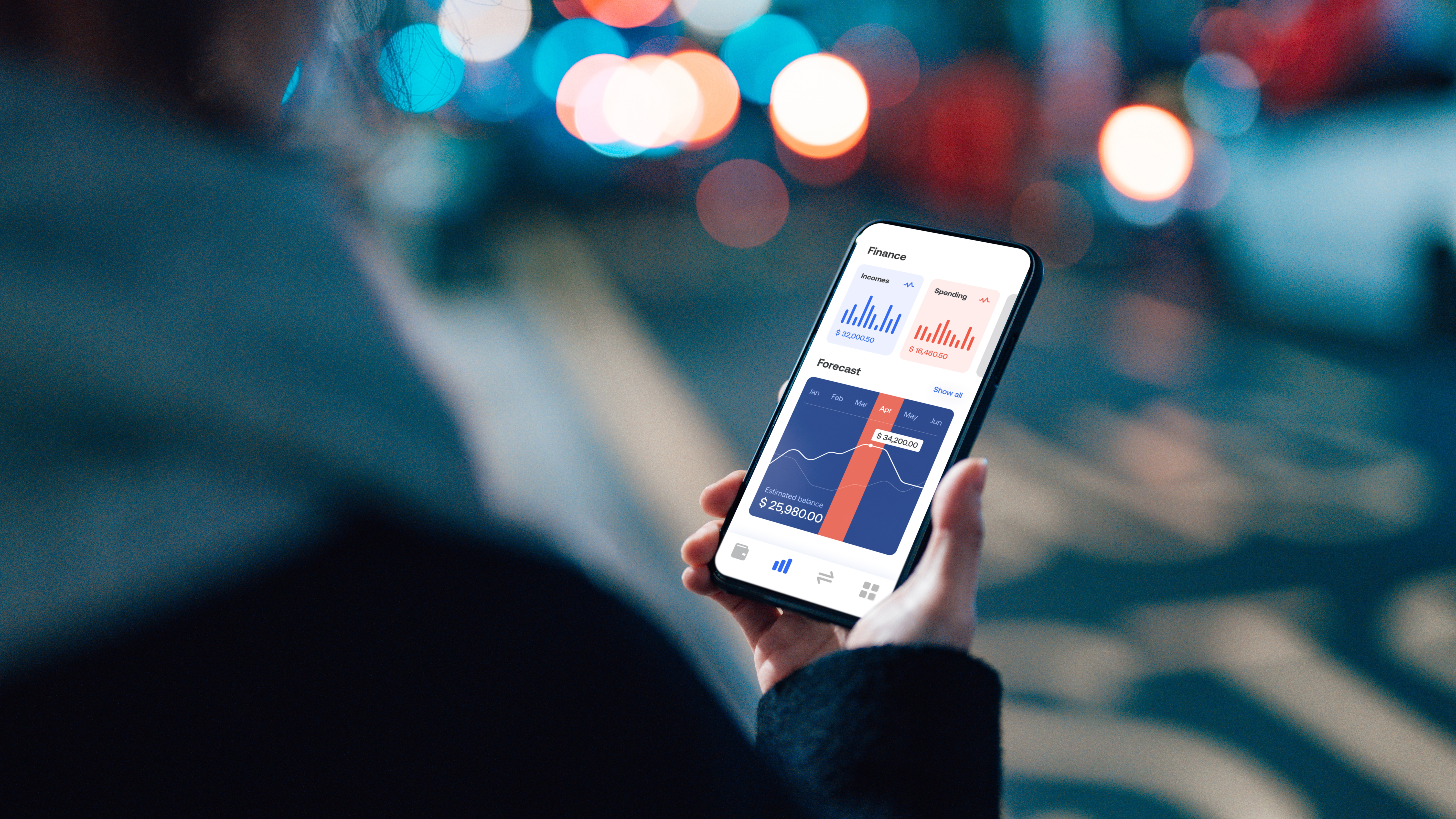
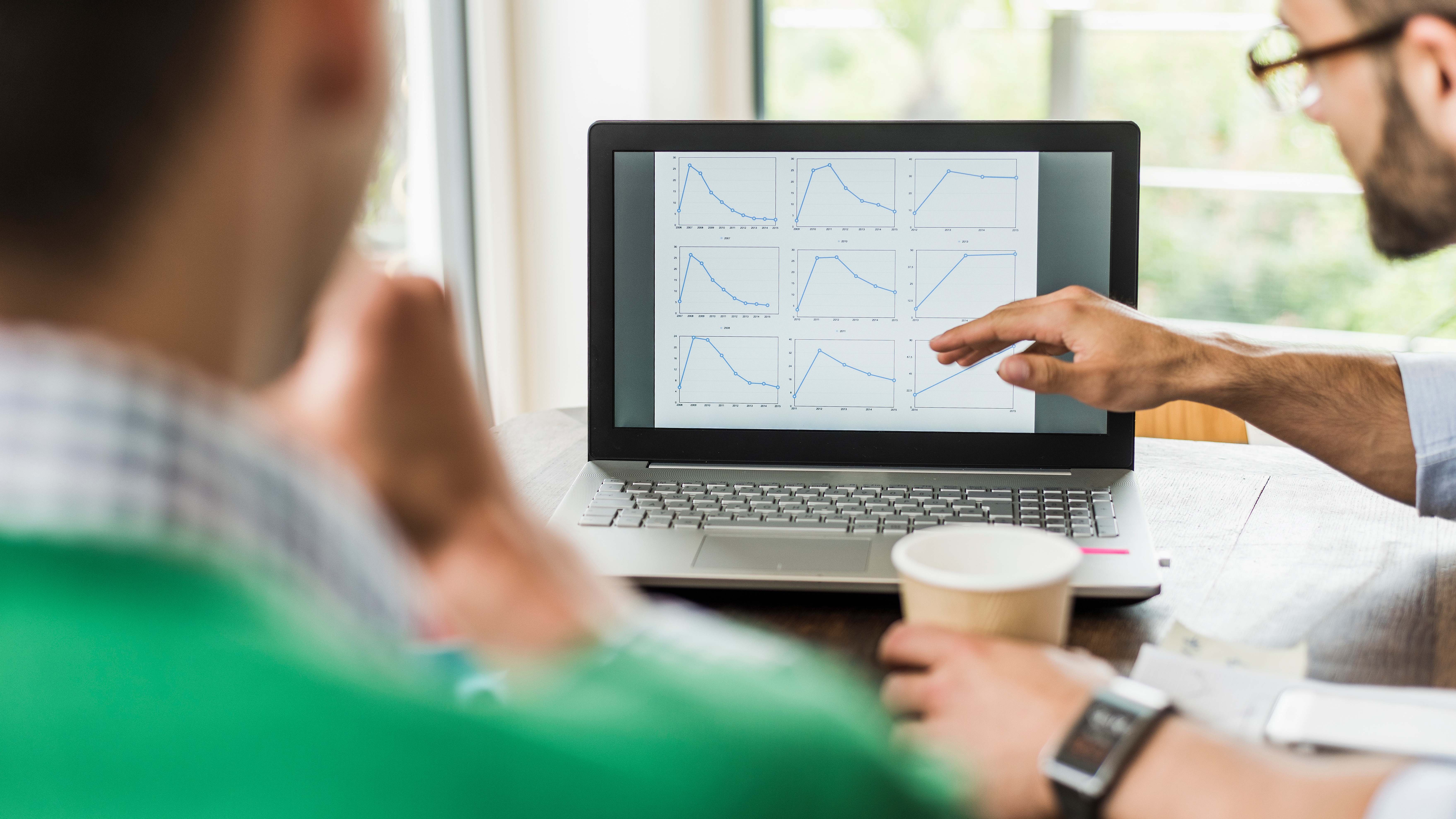
Retail motor insuranceAutomated machine learning approach for risk pricing and conversion rate modelling, impact analysis and what-if simulations |
Retail motor insurance
Conversion model using automated machine learning and adjusted commercial rate
Retail motor insurance
Risk modelling and machine learning for behavioural pricing including retention and conversion rate modelling
Homeowners insurance
Geospatial analytics and improved risk and technical pricing models
Tailored insurance pricing consulting
Our experts provide top-notch consulting for the insurance industry regarding product, pricing, underwriting and analytics. All our colleagues have a primary insurance background and in-depth consulting experience. They support you at every stage of our partnership – from initial concept to model development to scenario testing and commercial decisions.
Our big asset is our domain knowledge: we speak your language and understand your challenges. Whether we help you optimize your portfolio performance by improved control of your bottom line or whether we boost your top line growth – we aim for a multi-year impact.
Whitepaper Product & Pricing Day 1
Our pricing solutions for insurers and insurtechs
Strategy and portfolio management
- We advise on strategic choices, scale up your business and help set up pricing and underwriting committees. We support insurtechs and digital players to define the best rating strategy, portfolio steering frameworks and pruning actions.
- We help you build or grow your pricing team, we train your pricing specialists and provide them an independent and global view on insurance pricing and underwriting trends. We advise on competitive benchmarks leveraging the global exposure of Munich Re.
- We gain a holistic understanding of your portfolio by taking a deep dive into your historic data. Together with you, we build the perfect framework to steer your book towards prudent and profitable growth. This includes identifying, structuring and cleaning the data as well as enriching it with proprietary and external data.
Risk, demand and market modelling for decision-making
- Technical pricing: we help you develop the most accurate constrained and unconstrained cost-based models. We adopt traditional generalized linear/additive modelling (GLM/GAM) and expand into ensemble techniques and automated machine learning (AutoML).
- Behavioural pricing: based on granular market premiums information, we develop market models to cater to your diverse needs. We leverage the most advanced modelling techniques to build and integrate market predictions into your demand models. We also develop a competitive market analysis to get your first tariff segmentation right. Accounting for customer behaviours, including mid-term cancellations, endorsements and adjustments, is key to stay ahead of competitors. We help you leverage randomised price-tests to account for policyholder’s elasticity.
- Price optimisation: we set up impact / dislocation analysis, we run simulations and what-if scenarios to optimise ratemaking choices. Moreover, we advise on discount strategies in a data-driven approach. If market conditions allow, we can set up hard price optimisation including a revision of caps and collars to find the optimal individual price per customer at the point of sale.
Product development and underwriting
- We support you to revise existing products or conceptualise and design new ones, be it from scratch or by leveraging your data.
- We offer off-the-shelf products to cater e.g. for the enhanced needs of electric mobility or gap protection covers.
- We revise underwriting selection criteria and exclusion rule and advise on the adoption of pruning actions or improvement of the renewal strategy.
Advanced analytics
- Geospatial analytics: with microzoning solutions, we help you detect spatial patterns and model deficiencies and – when applied in pricing – improve your geographical risk segmentation. We help you boost your risk pricing of home and motor books, engineering new predictive features.
- Vehicle grouping: different dimensionality-reduction and clustering techniques allow us to develop vehicle-specific groups, leveraging advanced analytics and proprietary data enrichment.
- Electric Vehicle Analytics (EVA): a specific vehicle grouping solution for EVs, leveraging Munich Re’s exposure and advanced machine learning (ML) techniques. We developed sound statistical analysis that enables you to identify and classify vehicles according to their similarities. We validated the assumption that similar vehicles share a similar risk level, which translates into an easier assessment of (new) vehicles.
Contact us
Learn more about our consultancy on product, pricing, underwriting and analytics
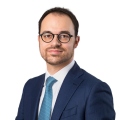
/Burlon_Davide_rgb_1-1.png/_jcr_content/renditions/original./Burlon_Davide_rgb_1-1.png)
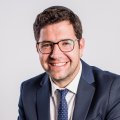
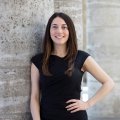
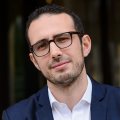
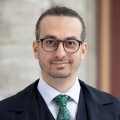