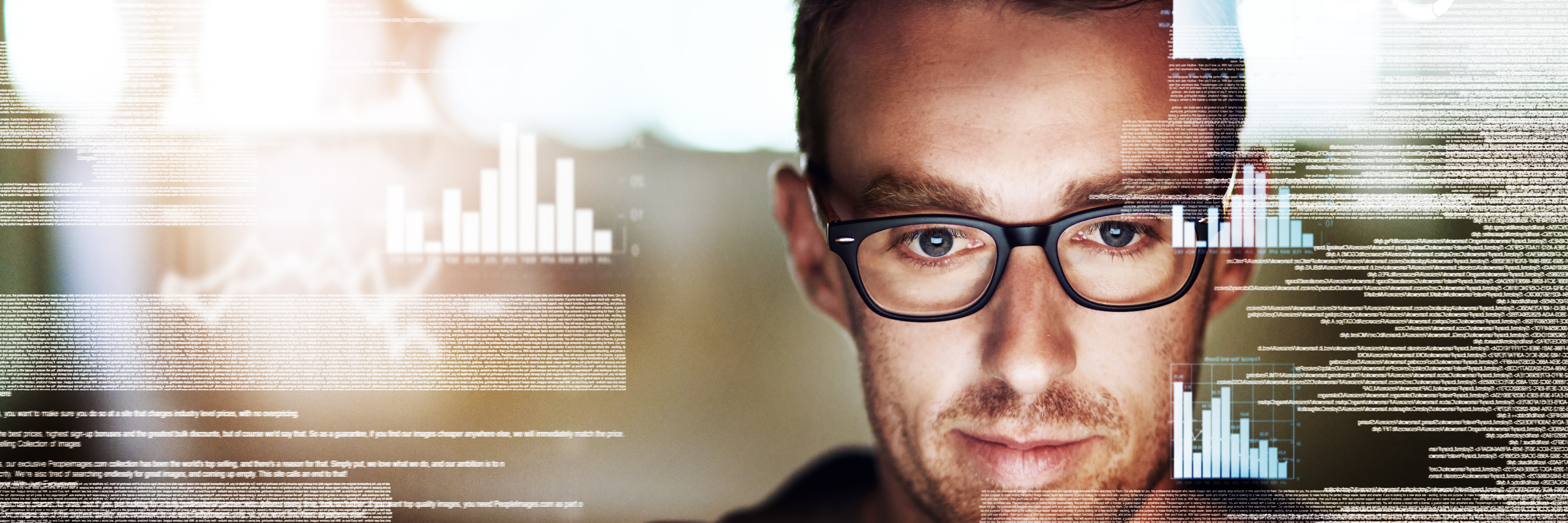
properties.trackTitle
properties.trackSubtitle
The LexisNexis® Risk Classifier with Medical Data score effectively stratifies mortality risk across age groups and is more effective at identifying high mortality risk when medical history is available.
Executive summary
Risk Classifier with Medical Data is a proprietary scoring algorithm that combines behavioral attributes and medical data to predict the mortality risk of individuals relative to other individuals of the same age and gender.
In 2020, Munich Re assessed LexisNexis® Risk Classifier version 2.0, a score based on behavioral and credit attributes. We concluded the scores are predictive of mortality in U.S. insured populations. That same year, Munich Re assessed ExamOne HealthPiQture™, a score based on prescription history, clinical lab testing results, and diagnosis codes from medical claims. We concluded the scores effectively segment mortality risk.1 Since then, LexisNexis Risk Solutions has collaborated with ExamOne to produce Risk Classifier with Medical Data. Carriers using these combined scores typically establish thresholds and decision guidelines to better streamline their underwriting process while managing mortality risk.
The Risk Classifier with Medical Data score is only provided for an individual with a combination of both behavioral data and medical data. In the absence of a medical hit, the model will return a score based on the behavioral only attributes.
Munich Re performed an analysis of Risk Classifier with Medical Data and confirmed it is predictive of mortality for the data set provided. The Risk Classifier with Medical Data score more effectively stratifies mortality risk across age groups. Also, it is more effective at identifying high mortality risk when medical history is available.
Risk Classifier with Medical Data also more effectively stratifies mortality risk compared to individual Risk Classifier and HealthPiQture scores.
Based on the data set provided, Risk Classifier with Medical Data shows evidence of greater mortality segmentation compared to Risk Classifier in an accelerated underwriting triage use case.
A company-specific insured population is not expected to have identical underlying characteristics as this dataset. Munich Re recommends life insurers interested in Risk Classifier with Medical Data conduct a study in order to calibrate the tool to the carrier’s own underwriting paradigm. This process will help carriers balance the score with the expected mortality of their unique target markets, distribution channels, and underwriting processes. For carriers who are already using a non-medical mortality score, Risk Classifier with Medical Data provides a more holistic assessment of mortality and greater risk segmentation due to its additional use of medical data.
Methodology
LexisNexis Risk Solutions provided Munich Re a dataset comprised of 2,189,608 depersonalized lives sampled from a combination of the U.S. general population and life insurance applicants. The data included Risk Classifier with Medical Data, Risk Classifier scores (both of which range from 0 to 997), and HealthPiQture scores ranging from -80 to 4900.
Each life entered the study between 2005 and 2019, with a significant majority of lives between 2012 and 2016, primarily from the general population. The latest study end date was in 2019. The final study population included 51,610 deaths. The expected mortality basis was an 85 percent non-smoker/15 percent smoker blend of the 2015 Society of Actuaries (SOA) primary select and ultimate, age-nearest birthday Valuation Basic Tables (VBT), primary select and ultimate age-nearest birthday tables split by age and gender with 1 percent historical mortality improvement.
Munich Re completed additional analyses of relative actual to expected ratios (relative risk) at various levels of age, gender, and HealthPiQture scores to assess the mortality risk stratification by Risk Classifier with Medical Data.
Results
Risk Classifier with Medical Data versus Risk Classifier
Figure 1 is a high-level comparison of the Relative Risk and distribution for Risk Classifier with Medical Data and Risk Classifier. Both scores are on a scale of 0 to 997, with higher scores indicating a better mortality risk and lower scores indicating a worse risk.
The Risk Classifier with Medical Data slope is steeper than the Risk Classifier, indicating more effective mortality segmentation. The Risk Classifier with Medical Data is more left skewed (median: 458) than the Risk Classifier (median: 641). A similar relative risk and distribution trend as in Figure 1 is seen at more granular gender, age, and HealthPiQture score levels.
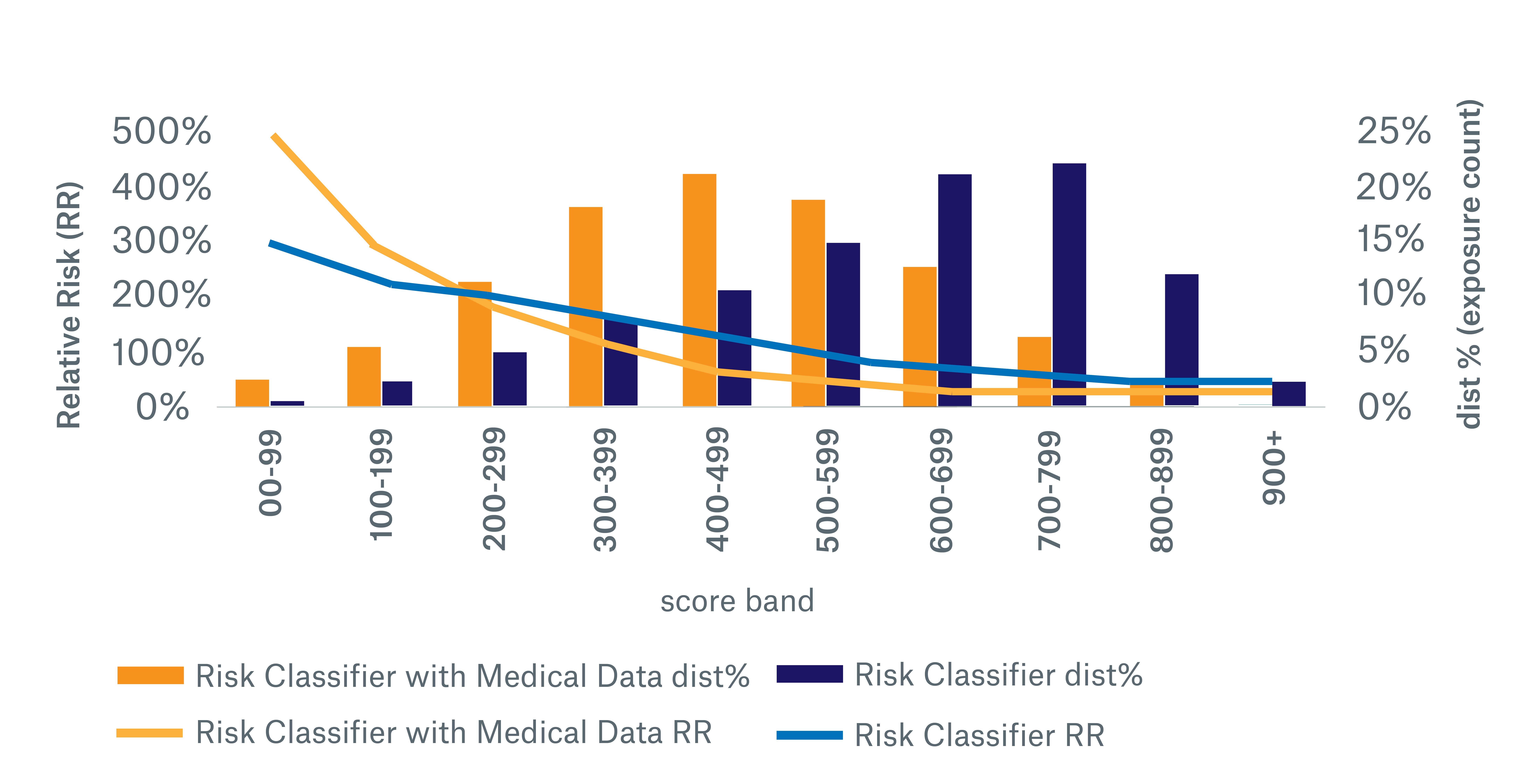
ExamOne HealthPiQture score
The relative risk and distribution for the HealthPiQture score, on a scale of -80 to 4900, is displayed in Figure 2. Here, lower scores indicate better mortality, and the mortality risk increases with higher scores. There is a noticeable spike at a score of 0. A similar pattern is observable at more granular gender and age levels.
Based on our prior HealthPiQture score assessment,1 among the three data components, we saw that clinical lab data is best at segmenting mortality, particularly at the highest mortality risk, compared to the prescription-only and medical claims-only scores.
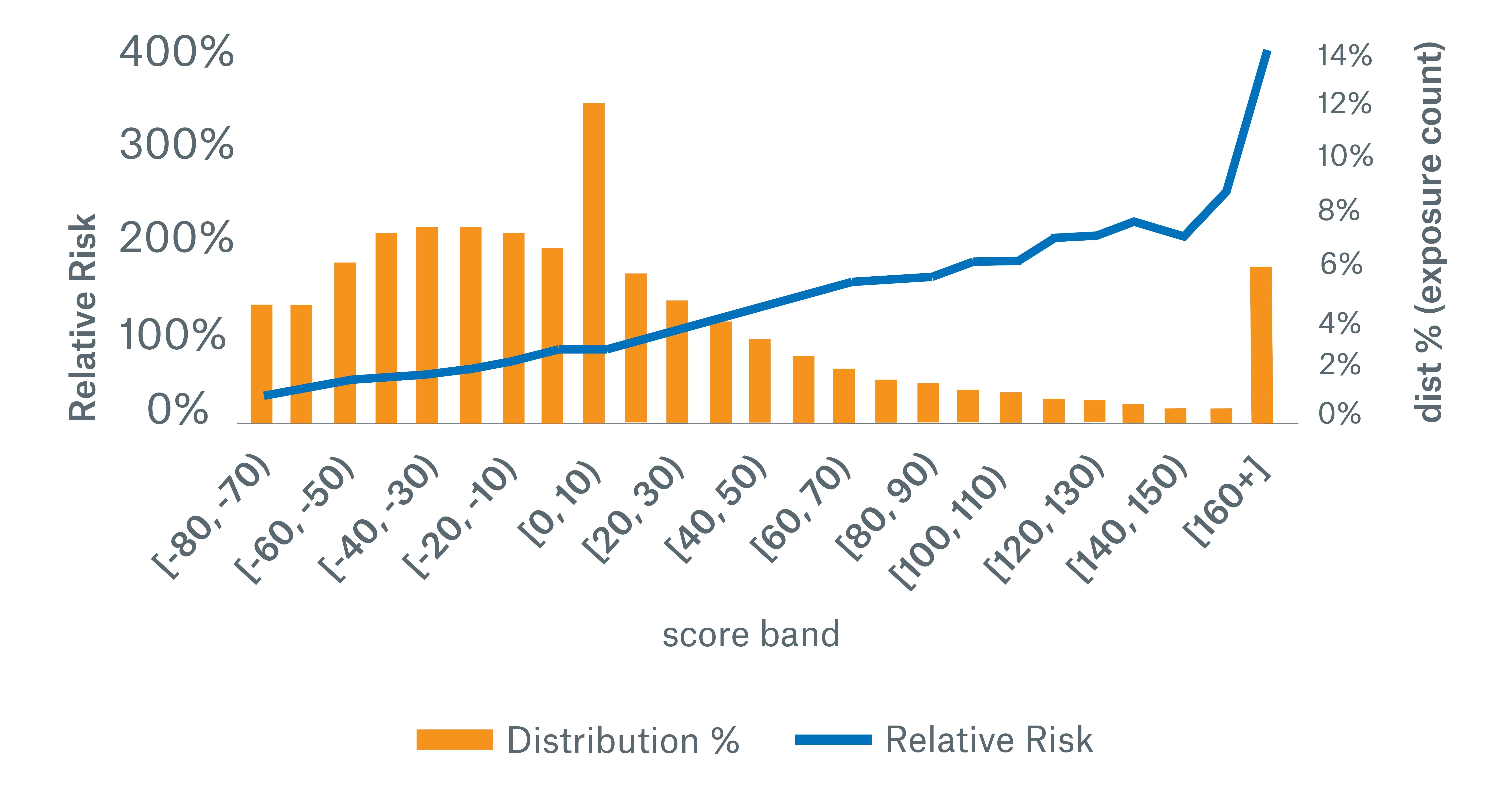
Behavioral, medical, and combination data
Figure 3 shows that the Risk Classifier with Medical Data score has a steeper slope relative to the individual Risk Classifier and HealthPiQture scores.
This supports the assertion that adding medical and behavioral attributes in the model results in better mortality segmentation than each of the individual sets of attributes by themselves.
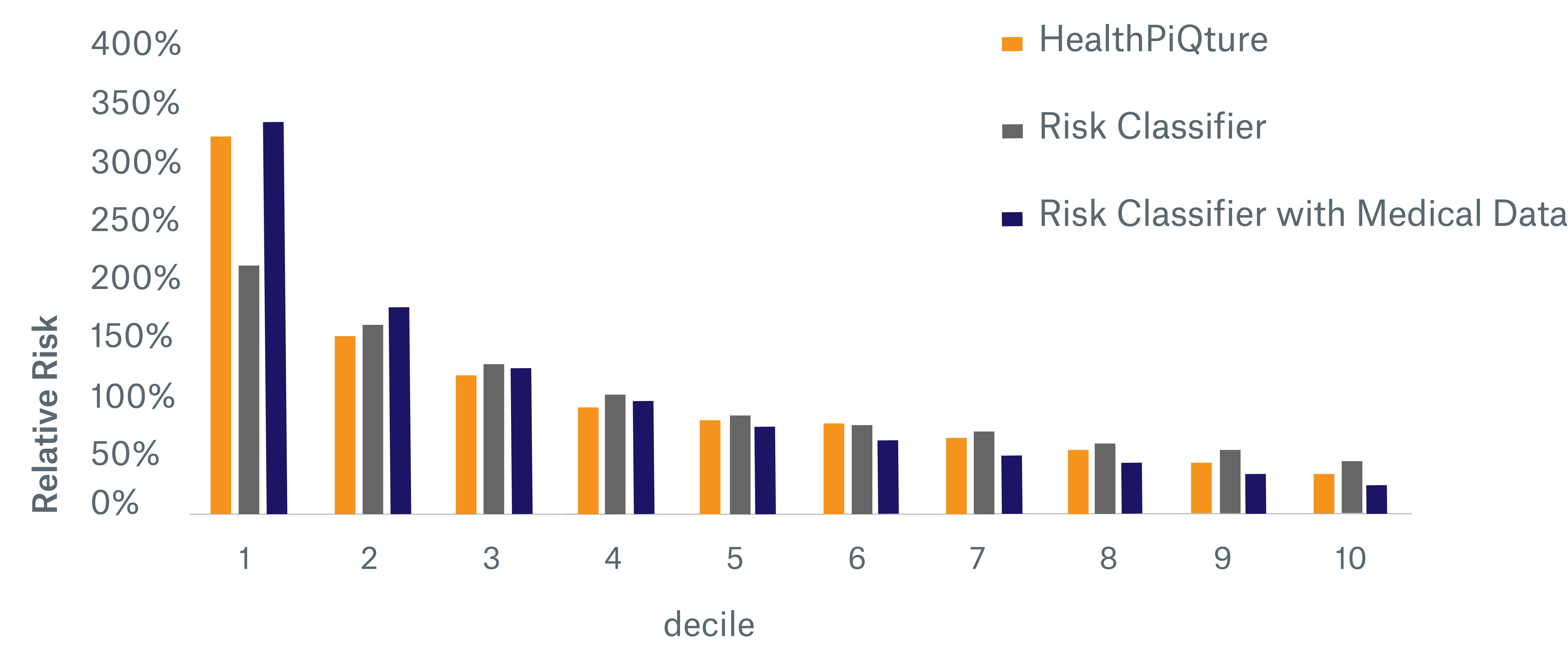
Three-way interaction of scores
Table 1 displays the relative risk and median HealthPiQture score percentiles (in parentheses) for different cell combinations of Risk Classifier with Medical Data and Risk Classifier scores, lower percentiles => worse median HealthPiQture scores.
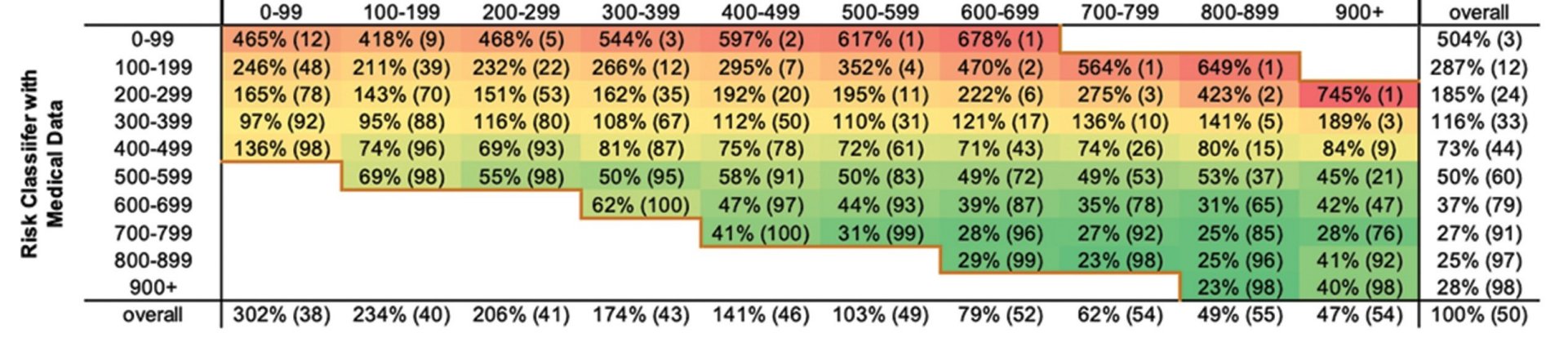
The worst mortality experience is observed in cells (top right corner) with better than median Risk Classifier score and very low (poor) median HealthPiQture percentile scores. Here, the favorable behavioral attributes are being outweighed by poor medical history resulting in high mortality for this subset. Based on our prior study of the HealthPiQture product, this may be driven by unfavorable clinical lab results, as we’ve seen this component among the three different HealthPiQture data sources to be the most effective at segmenting the worst mortality risk. For instance, an individual on low-risk prescription drugs and with good credit attributes may have clinical lab results that uncover evidence of an occult malignancy or alcohol abuse, for example.
Use case – accelerated underwriting triage tool
Figure 4 illustrates a potential use case for Risk Classifier and Risk Classifier with Medical Data scores to segment mortality risk into accelerated (AUW) and full underwriting (FUW) paths for an insurance applicant population. Both scenarios assume the same starting population and an identical straight-through processing (STP) rate of 50%. The relative risk (RR) differential between the two underwriting paths is greater using the Risk Classifier with Medical Data score, indicating better separation of mortality risk compared to the Risk Classifier score.
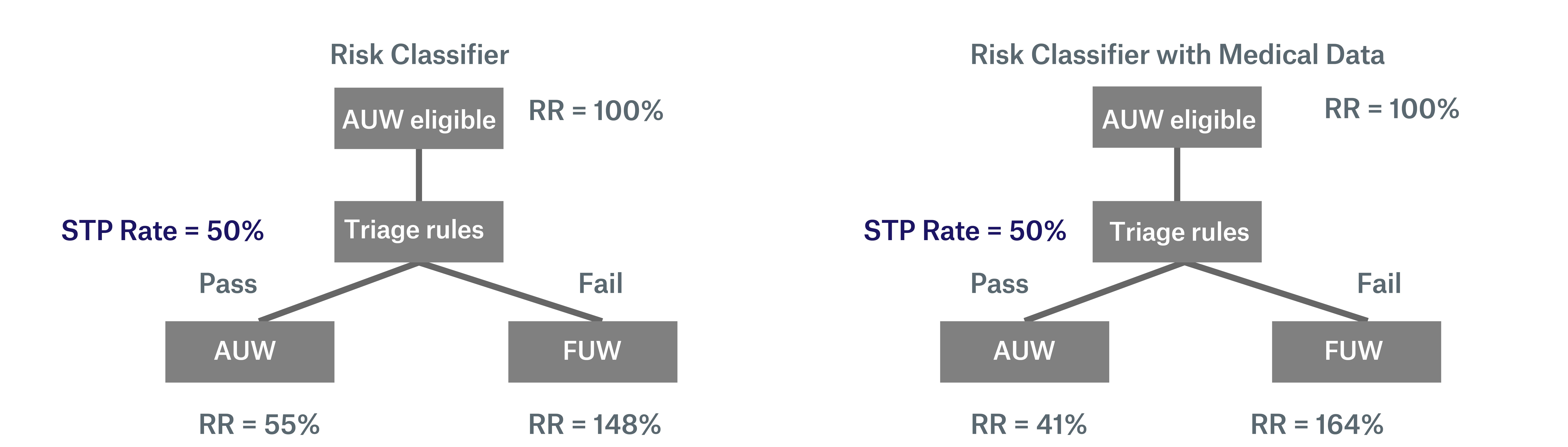
Age and Gender
Figure 5 shows the relative risk curves for Risk Classifier with Medical Data following a similar pattern across gender/age combinations.
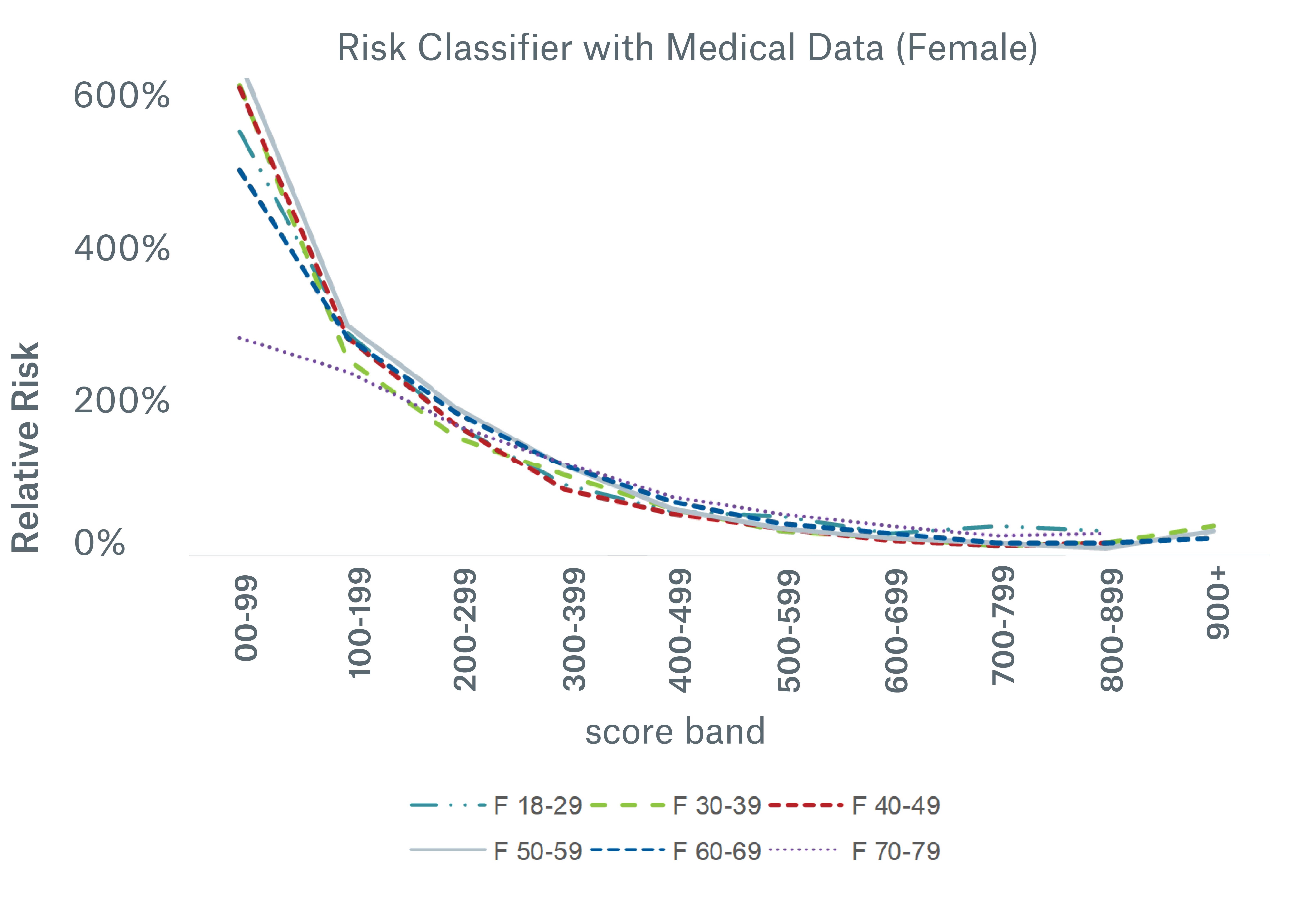
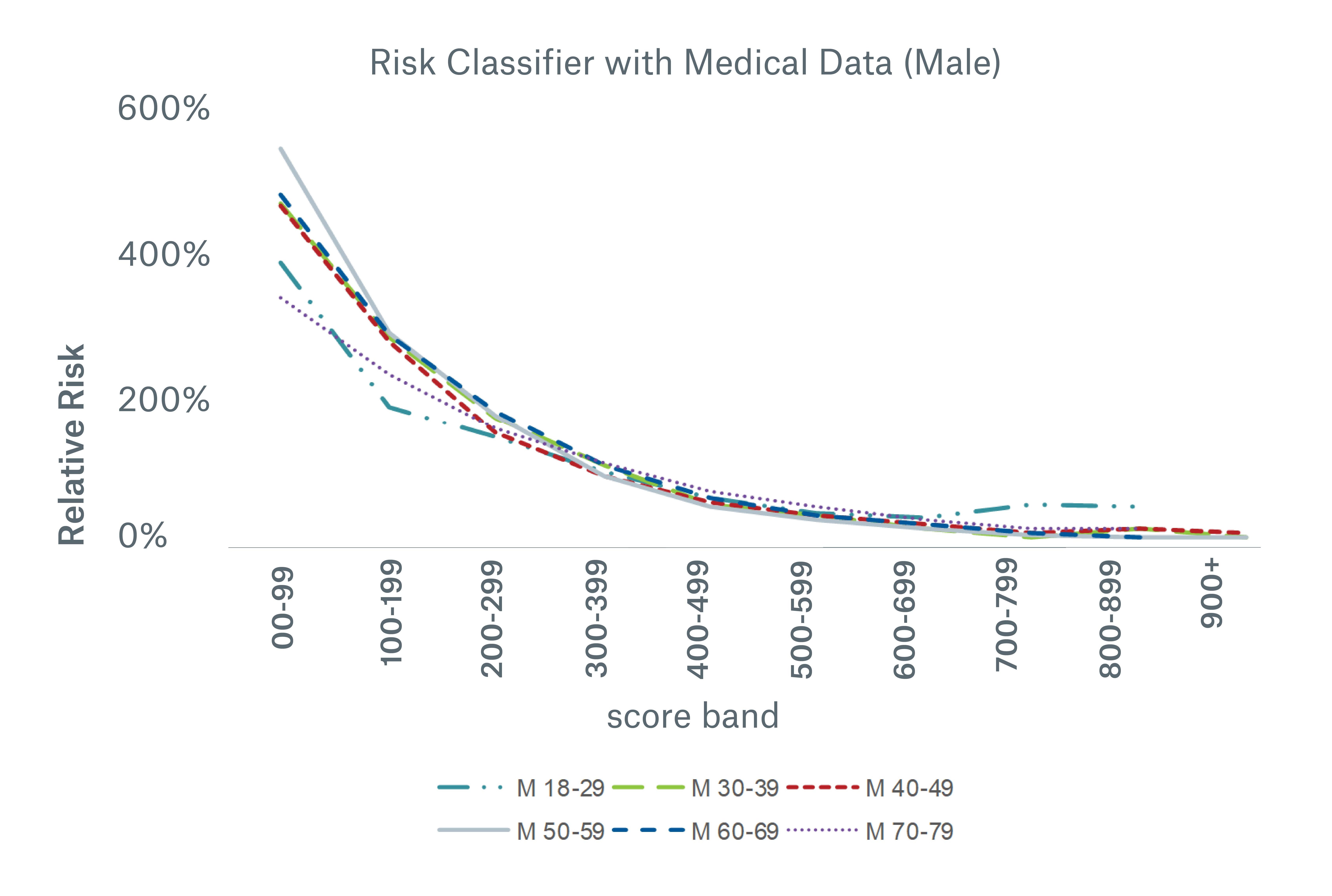
Distribution
The distribution of Risk Classifier with Medical Data scores across age group is relatively stable, as seen in Figure 6.

Summary
Munich Re concludes that the Risk Classifier with Medical Data is an effective predictor of mortality for the analyzed data set. Compared to the standalone Risk Classifier and HealthPiQture scores, Risk Classifier with Medical Data segments mortality risk more effectively across the range of the data. The leftward shift in scores for Risk Classifier with Medical Data means that carriers planning to implement this into their underwriting process need to recalibrate their score thresholds relative to what was used for Risk Classifier.
A score is provided for an individual with only behavioral data or a combination of behavioral and medical data. LexisNexis Risk Solutions will not offer a Risk Classifier with Medical Data score without a public record hit (i.e., based on medical data only).
In the context of use as an accelerated underwriting triage tool, Risk Classifier with Medical data provides better risk segmentation than Risk Classifier. In light of the COVID-19 pandemic, insurers have discovered an increased need for alternative data sources to underwrite applicants, and Risk Classifier with Medical Data could potentially play a useful role in this new underwriting paradigm. Even in a traditional underwriting process, Risk Classifier with Medical Data is especially useful when lab results are also accounted for in their HealthPiQture score.
As with all models incorporating a large number of data attributes and data relationships, considerations around how to reconcile model mortality assessments with traditional underwriting assessments should be closely analyzed and discussed with key stakeholders. These discussions should include those responsible for the final underwriting decisions and communicating those outcomes to the distribution or the proposed insured.
Finally, a company-specific insured population is not expected to have identical underlying characteristics to this general population; therefore, we recommend each carrier perform a study to best assess the value and application of Risk Classifier with Medical Data. Munich Re can assist carriers with the study, advise on changes to mortality assumptions, and recommend how to incorporate Risk Classifier with Medical Data in underwriting.
Download the report to view the whitepaperContact the author:

Related Content
Newsletter
properties.trackTitle
properties.trackSubtitle