
Accelerated underwriting:
Mortality slippage study and monitoring best practices
properties.trackTitle
properties.trackSubtitle
Introduction
Since the first accelerated underwriting programs came to market in 2012, these programs are increasingly viewed as the norm amongst life insurance carriers.1 For purposes of this article, accelerated underwriting (AUW) is defined as the waiving of traditional underwriting requirements (e.g., fluids and medical exams) for a subset of applicants that meet favorable risk requirements in an otherwise fully underwritten (FUW) life insurance process.
Accelerated underwriting programs, despite being in existence for a full decade, continue to lack the mortality data needed to effectively monitor experience that we have grown accustomed to in traditional underwriting programs. Although the industry is getting closer, credible AUW mortality experience has yet to emerge due to the fact that the majority of programs came to market in 2017-2018. At that time, carriers piloted their programs with limited accelerated business, but they have slowly ramped up over the past few years. In addition, AUW-issued business is disproportionately made up of younger and more preferred risks compared to traditional underwriting, so most successful programs have seen few actual claims to date.
In the meantime, carriers rely on AUW monitoring practices as leading indicators of future mortality experience to inform their decision-making. These monitoring techniques can vary in their data sources, timing, prevalence, and selection approach (i.e., random vs targeted). The two most common forms of random AUW monitoring include:
- Random holdouts (RHO) - Cases in the AUW workflow that are randomly selected for additional underwriting evaluation, typically through the FUW process and before a final underwriting decision and/or offer is made.
- Post-issue audits (PIA) - Audits conducted after an AUW decision and/or offer is made, most commonly by reviewing an attending physician statement (APS) or electronic health record (EHR). The risk class determination from this evaluation is considered a proxy for FUW.
Both holdouts and post-issue audits can be conducted on a targeted selection basis, in addition to random. Targeted audits provide additional protective value by focusing the review process on cases with attributes that are associated with higher-risk policies (e.g., a problematic sales agent, a poor-performing distribution channel, policies flagged by MIB F or Rx Recheck). However, because targeted audits are skewed towards cases more likely to be misclassified, they are not as useful for mortality monitoring. For that reason, the remainder of this article will be focused on the two leading random monitoring methods: Holdouts and post-issue audits.
Background/methodology
Munich Re Life US monitors AUW programs using random audit data provided by life insurance carriers, which allows us to estimate future mortality experience based on underwriting risk misclassification within the AUW decisioning process. As of year-end 2023, we’ve collected 11 years’ worth of RHO and PIA monitoring data on over 33,000 lives across 30 AUW programs with the goal of capturing emerging mortality trends in the U.S. individual life accelerated underwriting market. For purposes of this analysis, we have excluded any AUW program dataset with fewer than 50 cases.
We use this data to derive mortality slippage, which we define as the implied mortality load relative to FUW mortality due to risk class misclassification in the AUW process. It is calculated on a present value of future claims basis using Munich Re’s internal view on a program’s mortality for a given age/gender/risk class. Risk class misclassification refers to differing risk class outcomes between the AUW and RHO or PIA estimated risk class.
This article highlights emerging accelerated underwriting monitoring trends and shares some of our high-level mortality slippage findings.
Study findings
Misclassification trends

Mortality slippage trends
Across the life insurance industry, when comparing misclassification results of AUW programs that use one or both of these common monitoring methods, we’ve observed that RHOs generally result in lower overall mortality slippage compared to PIAs, even within the same programs. In aggregate, we estimate an overall mortality slippage of 12% from RHO cases vs 15% from PIA cases. The higher slippage seen in post-issue audits is likely a result of several factors:
- Additional medical history uncovered by an APS or EHR that often cannot be uncovered with fluid testing alone, leading to more severe misclassification from an APS or EHR review.
- “Reverse misclassifications” are more likely to be captured in RHO results vs PIAs, and result in negative mortality slippage. On average, we’ve observed nine times the prevalence of reverse misclassifications in the RHO results compared to PIA.
- Sentinel effect introduced by a random holdout process. This refers to the concept that applicants (or agents) will respond more truthfully if they are aware of a potential fluid-testing requirement.
- Withdrawals in response to the RHO selection process can help weed out applicants who may have not fully disclosed their health status. However, it’s important to monitor the level of RHO withdrawals, which if excessive, may result in a biased audit sample that is not representative of the entire AUW applicant pool.2
- APS/EHR hit rates could be skewed towards individuals more likely to visit a doctor, depending on the target market and age demographic. Therefore, it’s important to monitor the age/gender distribution of lives that make up a PIA sample to ensure it is representative of actual AUW-issued policies.
While we consider RHOs to be the best proxy for full underwriting, there are pros and cons to both auditing methods, as evidenced by the factors above. That’s why we believe the best practice is to do a combination of both monitoring methods to maximize your AUW program learnings!
Looking back at 11 years of AUW monitoring data, we have observed aggregate mortality slippage trending up over time. While this upward trend is partially influenced by an expanding pool of programs represented in our study over time, when isolating individual programs with recurring monitoring data over consecutive years, we still see more programs with an upward-trending slippage versus downward trending. The goal of a robust monitoring program is to identify gaps in the AUW decisioning process and continually improve the program in response to audit findings. However, the improvements in AUW decisioning can be offset by increased misrepresentation (“misrep”) that can occur with a changing applicant pool – a potential result of increased awareness around the fluidless underwriting path. Capturing the reasons for misclassification and their prevalence in the audit findings over time can provide valuable insights for carriers looking to understand the drivers behind their mortality slippage trends.
Beyond trends in the monitoring type, we’ve captured misclassification trends by policy-level characteristics through the seriatim de-identified random audit data we’ve aggregated and analyzed. The findings below are representative of 25 AUW programs (over 28k total audited cases) with available policy-level details.
Trends by policy-level characteristics
- Males are more likely to be misclassified in AUW programs, with over 1.25 times overall mortality slippage compared to females.
- Older issue ages are correlated with higher AUW mortality slippage, as their risk class assessment is more severely impacted by the removal of underwriting labs and exams. This upward trend is demonstrated in Figure 2.
- Increasing face amounts have a downward mortality slippage trend, as shown in Figure 3. This may be a result of more stringent AUW data requirements and more human underwriter review at higher face amounts, or could be driven by different target markets/demographics represented across the spectrum of face bands.
- Term products have nearly 1.8 times higher mortality slippage than perm products, as estimated by emerging misclassification results. While this also could be a result of differences in markets and/or demographics, it’s worth noting that perm products are much less prevalent than term products in AUW, representing just 10% of our total study dataset.
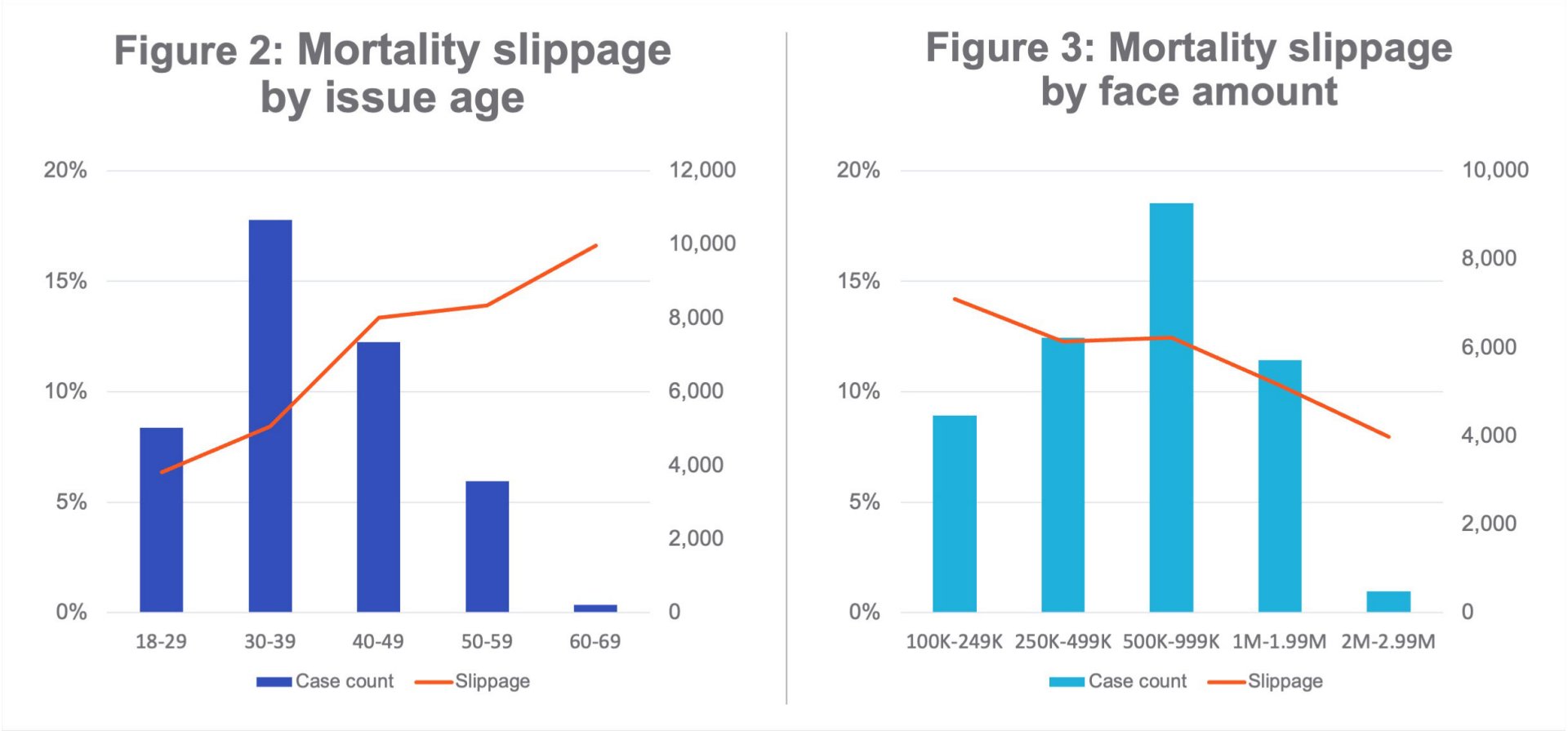
Tobacco misrepresentation
Tobacco misrepresentation is defined as the number of non-disclosed smokers as a proportion of the number of true tobacco users (self-admitted and undisclosed) in the audit sample. Based on our cumulative monitoring dataset, which includes over 1,400 confirmed tobacco users, we are seeing the following trends:
- Overall tobacco misrep is currently at 40%, based on the aggregate number of confirmed tobacco users in the dataset across all 30 programs.
- Random holdouts are more successful at uncovering tobacco nondisclosure than post-issue audits, with 1.5 times higher tobacco misrep in RHO versus the PIA monitoring data. This is likely a direct result of insurance labs routinely testing for cotinine versus APS or EHRs, where doctors may not always document or even be aware of a patient’s tobacco usage.
- Males have nearly 1.2 times higher tobacco misrep than females.
- Tobacco misrep has been trending up over time when we look at aggregate tobacco misrep across our entire dataset, likely driven by the changing mix of carriers and the continual addition of newer AUW programs into our study. However, when isolating individual programs with consecutive years of monitoring data, we see a relatively equal split between the number of programs experiencing an increasing versus decreasing tobacco misrep trend.

Best practices
There are several best practices that carriers can implement while monitoring their AUW programs, keeping in mind that estimated mortality slippage is only an indicator of future mortality experience and not based on actual claims experience.
- Start broad and scale down – It takes time for a credible number of RHO or PIA cases to develop. Carriers that want to acquire learnings quickly will start with a high percentage of cases monitored, sometimes even 100% when they are in pilot phase. Once carriers are comfortable with the results, they can scale back to a more manageable monitoring level. Another reason for selecting more cases than intended to audit is to account for withdrawals in response to RHO selection, and not all cases requesting post-issue APS or EHRs will be returned.
- Have a well-documented monitoring process and procedure in place – Monitoring data should be reviewed and evaluated by an experienced underwriter with the scrutiny as if the underwriter were evaluating the risk as new business. Target versus actual percentage of RHO and/or PIA cases, rescission thresholds, magnitude of rate differences, and source(s) and reason(s) for rate differences should all be noted. Also, if there is a rate class difference but rescission is not pursued, a file should be documented to prevent re-issue or reinstatement (without additional investigation).
- Utilize available tools – While MIB Plan F and APSs are widely used, EHRs are emerging as a valuable PIA tool. Furthermore, prescription or medical claims histories can be utilized as stand-alone PIA tools or to triage APS or EHR reviews.
- Be agile – Ongoing monitoring of programs can provide insight into pockets of business that may be experiencing higher misclassification and/or attracting anti-selection. Being able to react quickly to monitoring findings and adjusting program underwriting on the fly will allow you to close these loopholes before actual mortality experience begins to emerge.
- Leverage your partners – Monitoring mortality slippage is a team effort. Engage your distribution and reinsurance partners in the process and sharing of results. Munich Re shares the results of our detailed carrier-specific monitoring analyses back with clients to share trends and high-level insights on their individual program. We can work with you to strengthen your AUW programs.
Summary
The lack of credible mortality data in still-evolving AUW programs means that carriers must continue to rely on AUW monitoring as an indicator of future mortality experience. Our study of AUW monitoring data found that an average program can expect to see mortality slippage in the 10%-15% range. While random holdouts generally result in lower overall mortality slippage compared to post-issue audits, using a combination of both monitoring methods could maximize AUW program learnings. Across both monitoring methods, most (81%) randomly audited cases are assessed at the same risk class as the AUW decision. Aggregate misclassification trends by policy level characteristics signal that males, older issue ages, and lower face amounts have higher mortality slippage. Tobacco misrepresentation remains a particular area of concern for AUW, and continuing to find solutions to combat this increasing misrep trend will likely continue to be a focus of the life insurance industry.
Munich Re has the expertise and experience with tools that can support carrier AUW programs. We have identified several AUW monitoring best practices, including starting with broad monitoring and scaling down as comfort grows, utilizing available tools, and developing agile procedures for quick reaction. As a follow-up to this paper, we’ll take a deeper dive into existing gaps in AUW programs and what can be done to close them.
/Lisa%20Seeman.jpg/_jcr_content/renditions/original./Lisa%20Seeman.jpg)
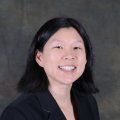