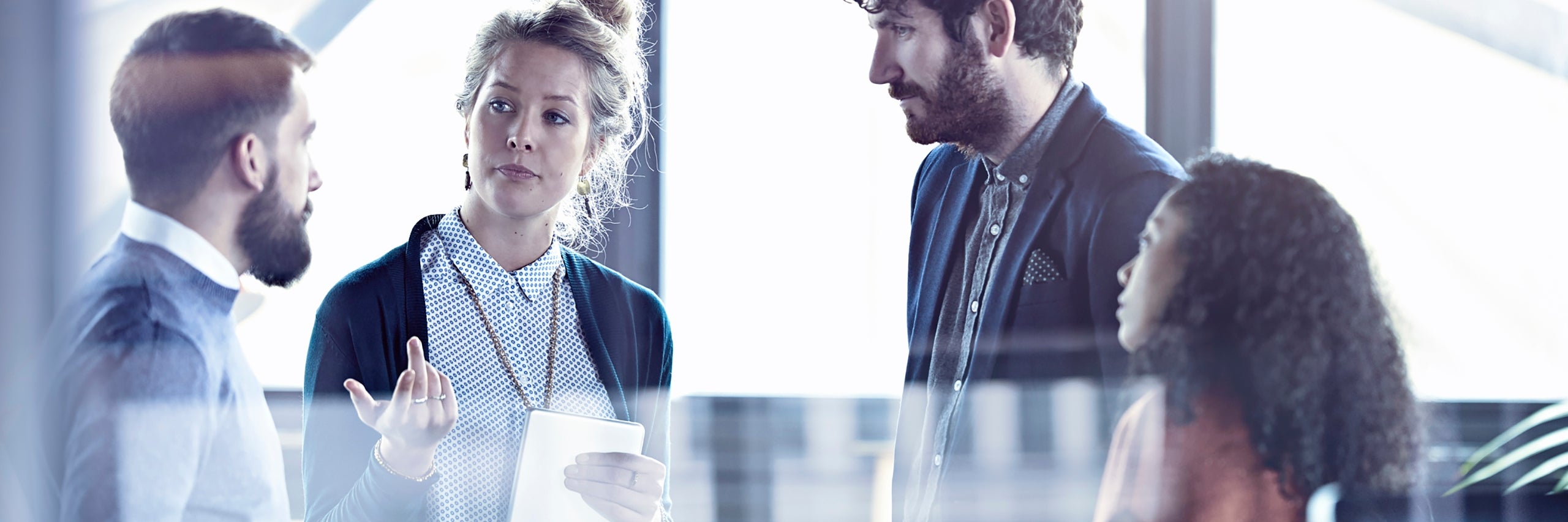
Analyzing Individual Life Insurance Mortality Trends - Socioeconomic Status Impact
properties.trackTitle
properties.trackSubtitle
Munich Re’s North American biometric research team continually monitors industry experience (mortality, morbidity, lapses). We hope to stimulate discussion with our business partners and across the insurance industry by sharing our key findings. We believe these discussions will lead to a better understanding of emerging experience and its importance in assessing underlying risk.
This paper is the second in a series taking a deep dive into analyzing the U.S. population mortality trends. The first paper published earlier this year discussed the impact of Cause of Death (COD) prevalence in analyzing mortality improvement (MI) trends for the life insurance population. In this second paper, we compare and contrast two methods to segment the population’s mortality experience to account for the socioeconomic status (“SES”) effect of the life insurance population, and discuss some of our insights from this analysis.
The population mortality data we analyze in this paper is publicly-available data published by the Centers for Disease Control and Prevention’s (CDC) Wide-ranging Online Data for Epidemiologic Research (WONDER) database, released in December 2017.
The Socioeconomic Effect
It is well-known that the U.S. population mortality experience can be distinctly different between SES, with a widening gap between the rich and the poor.1 When analyzing the U.S. population data to determine mortality trend assumptions for life insurance, it is important to account for the SES effect because the life insurance population is heavily skewed towards the more affluent population.
The challenge with segmenting mortality experience by SES is that key indicators such as income, net worth, and education attainment are not always available or accurate on death certificates. Alternatively, there are approximation methods that can be used to segment the population by SES, we will discuss two approaches in this paper: county- level income, and education attainment.
County-Level Income
The first method is to segment the population mortality data by county using county-level income. Under this approach, we ranked all U.S. counties by historical Per-Capita Income (PCI) published by the U.S. Bureau of Economic Analysis, and analyzed mortality data from CDC WONDER by percentile of county-level income.
A similar approach was used in a paper published by the Society of Actuaries (SOA) in January 2019, titled “U.S. Population Mortality Observations – Updated with 2017 Experience". 2 Observations from the SOA paper illustrated a clear difference in both overall mortality level and mortality trends between the top 15% vs. the bottom 15% by county income.
The main limitation of this approach is that most counties do not have a uniform distribution of income within their population; in fact, some counties in the US have extreme wealth disparity that leads to 20-30 years3 of life expectancy difference. Categorizing by county-level income can misclassify individual deaths and exposures that have a different income profile than the rest of the county.
Education Attainment
Another approach is to proxy SES using education attainment levels. Education has been included as an element of death certificates in most U.S. states since 1989 and is available in the Public Use Mortality Data published by CDC. Additionally, the total population estimates (i.e., the exposure) by education attainment can be retrieved from the U.S. Census Bureau. Combining these two sources allows us to conduct mortality studies for the U.S. population by different education attainment levels.
One challenge with this approach is the data quality of education attainment level on the death certificates, which is often missing or incorrect. We attempted to address this issue using a paper published in May 2010 by B. Rostron, J. Boies, and E. Arias, “Education Reporting and Classification on Death Certificates in the United States” (Vital and Health Statistics 2(151)). In this paper, the researchers used self-reported educational attainment from the 1992-1998 Current Population Survey (CPS) Data that was included in the National Longitudinal Mortality Study (NLMS). This allowed the researchers to determine which study participants had died by the end of 1998 and identify the educational status reported on the death certificate. Not only did this highlight the proportional frequency of misidentification, but also the appropriate relative educational proportions of those who have no educational information listed on the death certificate.
We used the results of the paper to create a misclassification matrix of educational attainment to redistribute the records from CDC WONDER with unknown education fields. This allowed us to divide the U.S. population into four education attainment segments, which became the “exposure” in mortality rate calculation for each of the following segments:
- No high school degree
- High school degree
- Some college but no Bachelor’s degree
- Bachelor’s degree or more (includes Masters, PhD, etc.)
Proxy for Insured Population
Using the county-level income and education approximation methods above, we compared the life insurance population’s mortality (approximated by industry table VBT15 aggregate ALB ultimate), to the mortality of the following 4 sub-segments of U.S. population:
- Population with Bachelor’s degree or higher education (BA+)
- Population with less than Bachelor’s degree (BA-)
- Population from top 15% income counties (Top 15%)
- Population from bottom 15% income counties (Bottom 15%)
Graph 1: U.S. Population Mortality by SES
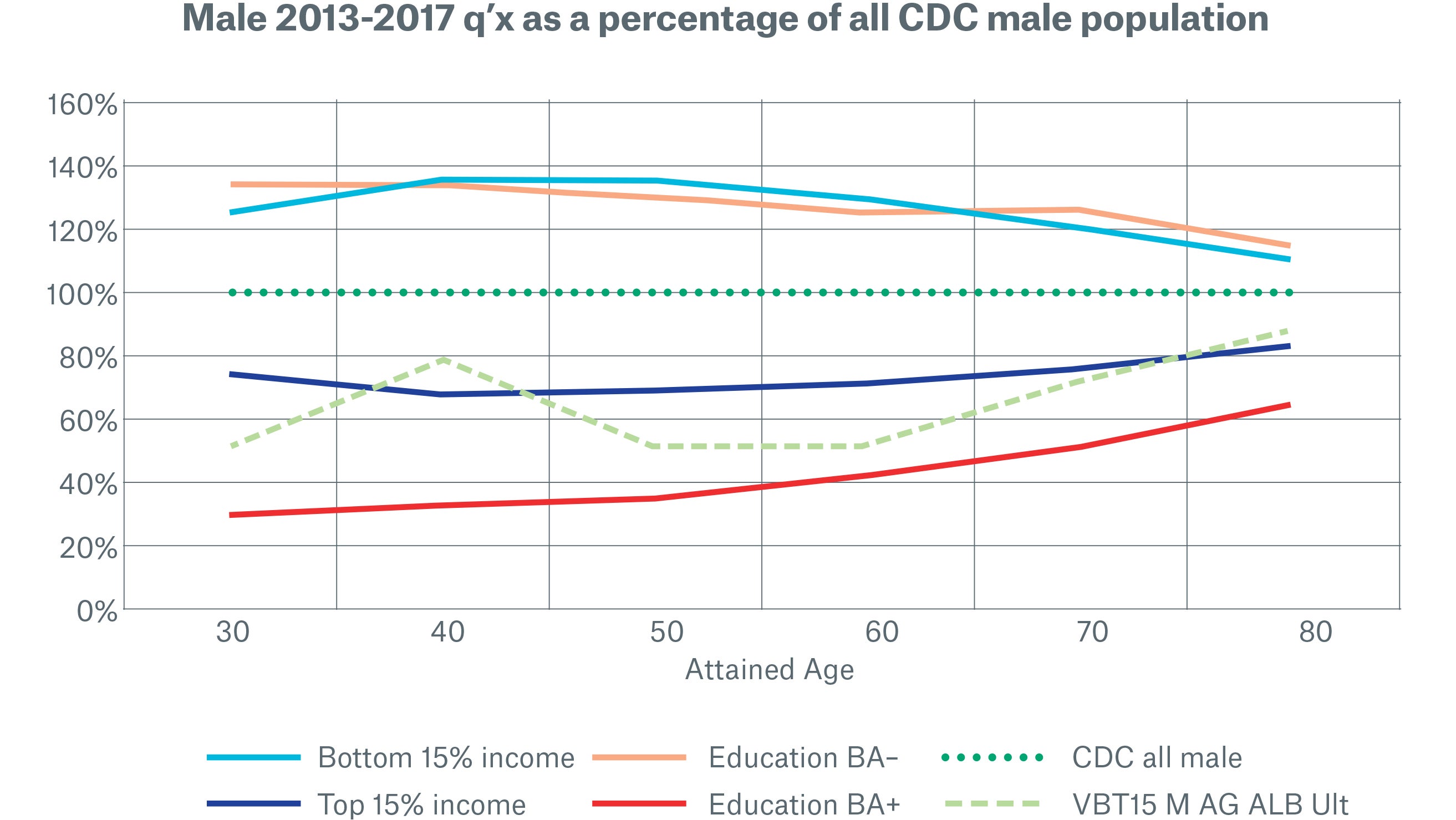
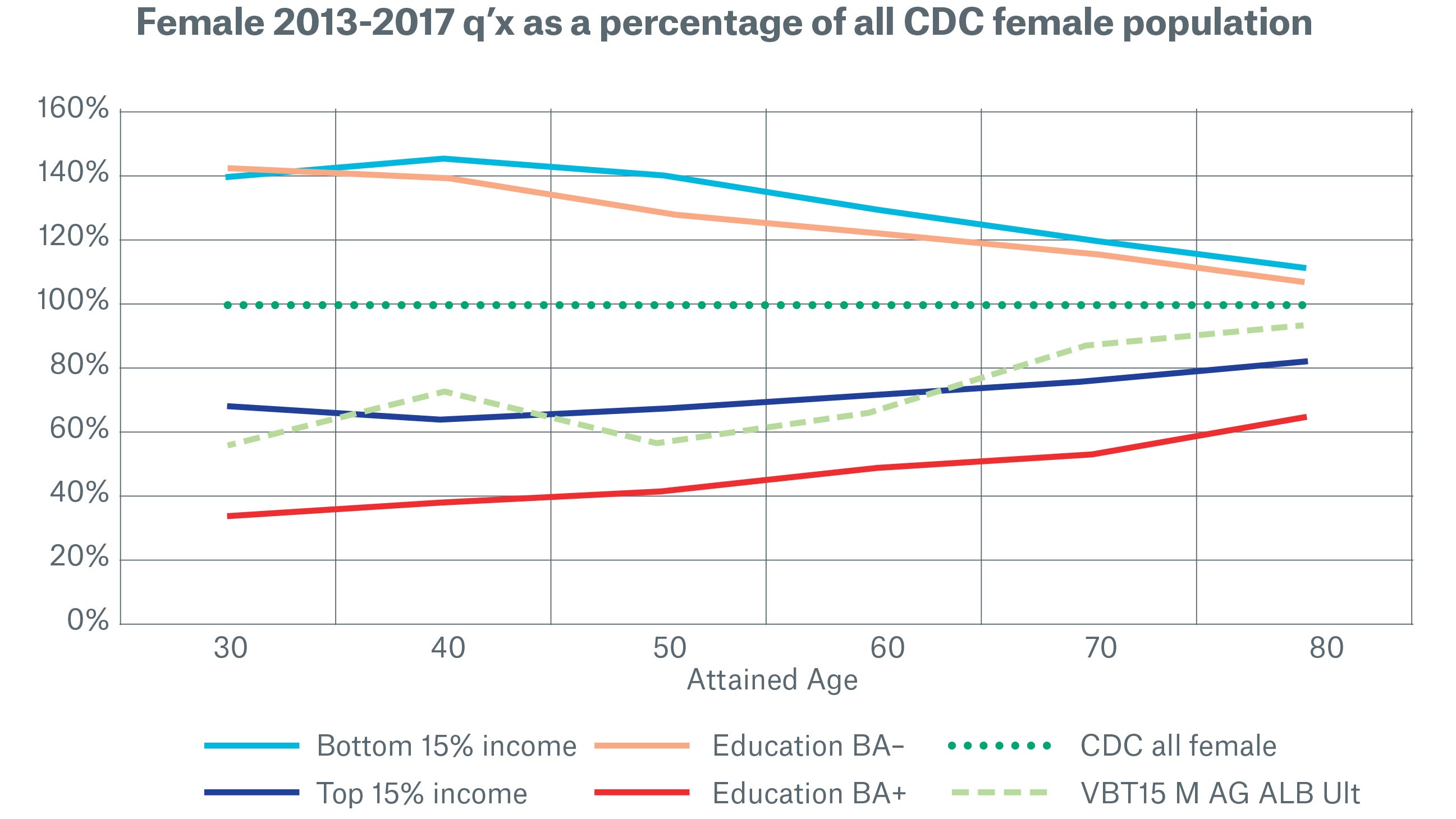
From Graph 1 above, the following observations were made:
SES mortality gap - Both county income and education attainment can distinctly segment U.S. population’s mortality.
The mortality gap is wider at younger ages - The mortality gap between SES is generally wider at younger ages and narrower at older ages. The one exception is that under the county income approach, the gap appears more narrow at age 30 than age 40. It is possible that this is due to limitation of the county-level approach described above, as the exception is not observed under the education attainment approach.
Education attainment provides a stronger segmentation - Population with education attainment of Bachelor’s or higher has even lower mortality than those from the top 15% income counties. If we wanted to further increase the segmentation power under the county income approach, it is possible to use a higher percentile (i.e., top 10%), but at the expense of reducing data points and credibility.
Higher SES is a proxy for life insurance population - For most attained ages between 30-70, the insured population’s mortality (proxied by VBT15) is between or close to the “Top 15% Income” and “Education BA+” mortality. This indicates that U.S. population with higher income or education can be a good proxy for life insurance population, and analyzing long term mortality trends for the higher SESs may help approximate mortality trends for the life insurance population.
Mortality Improvement Trend Observations
Using the income and education methods described above, we compared MI trends for the four segments of the U.S. population (top 15%; bottom 15%; BA+; and BA-). The average annual MI rate was calculated for each subgroup, over both the long term (2000-2016) and the short term (2011-2016).
By county income (male and female combined):
Graph 2: US Population Annual MI Rates by County Income
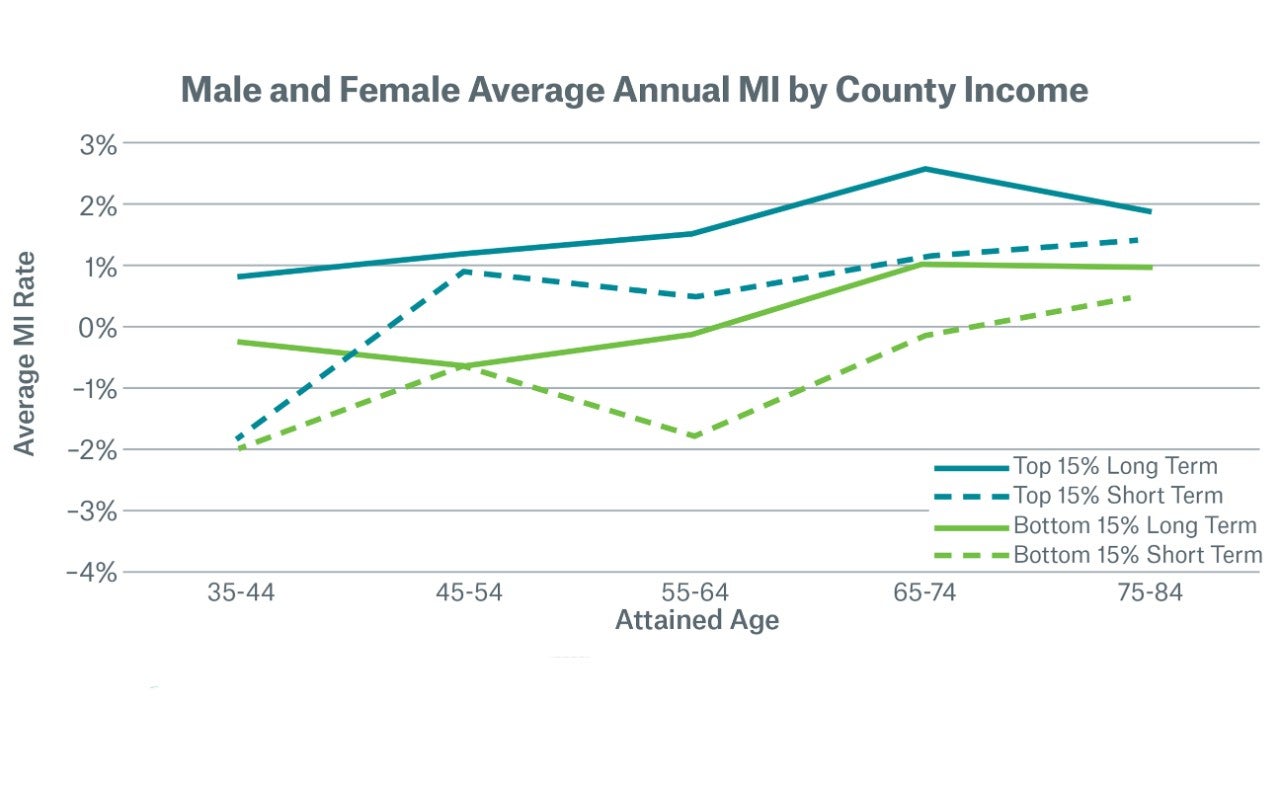
Graph 3: US Population Annual MI Rates by Educational Attainment
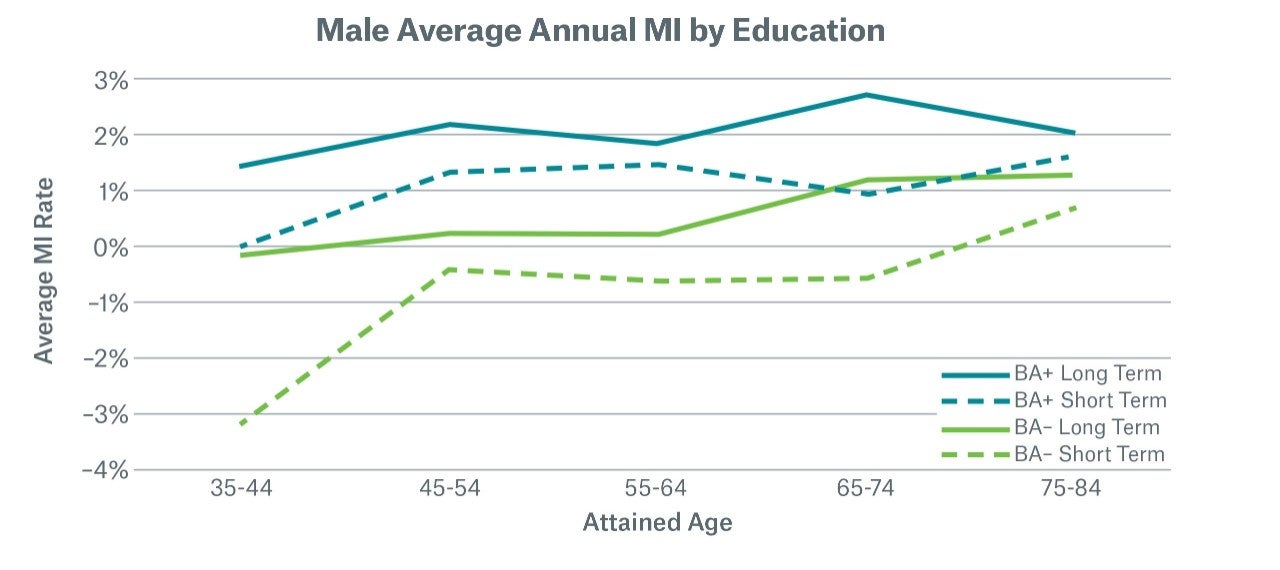
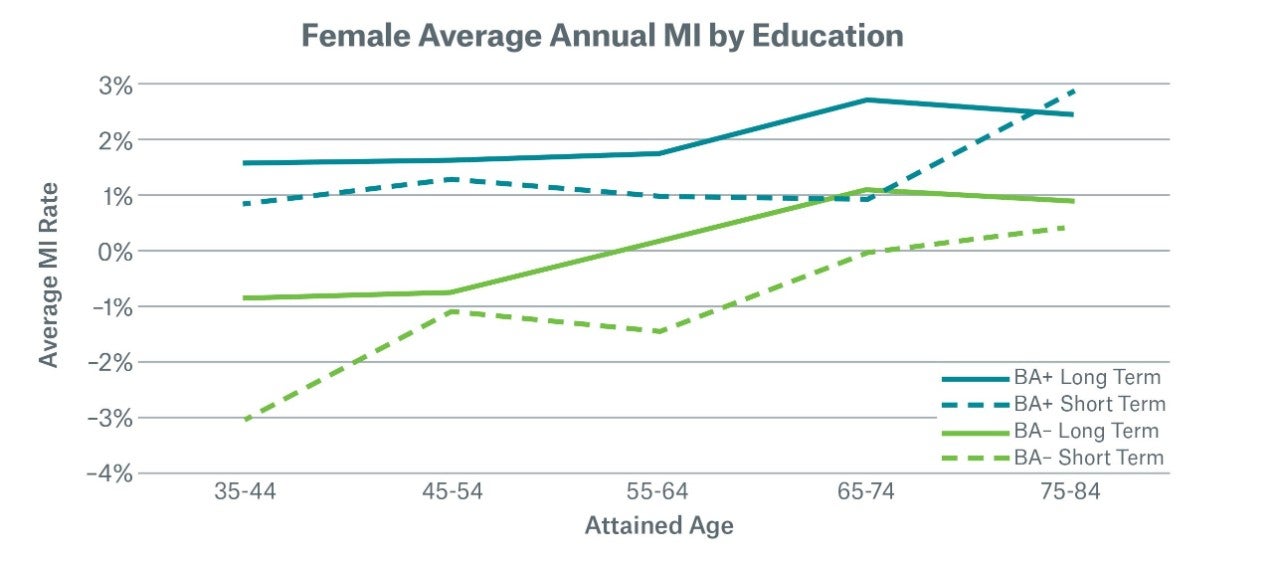
From graph 2 and 3, we observed the following:
Mortality improvement is faster for higher SESs - The higher SESs (BA+ and Top 15%) showed significantly higher MI than the lower SESs (BA- and Bottom 15%) both over the long term and the short term. This observation further validated the widening mortality gap between the rich and the poor in the US.
Difference in mortality improvement is across all ages – The MI difference between SESs is distinct across all ages in both study periods. The only exception is at age 35-44 under the county income approach, where the short term MI is similar for the top and bottom 15%. It is possible that this is due to the limitation of county income approach, because the same observation cannot be made under the education attainment approach.
Bachelor’s or higher educated population has similar or better mortality improvement than that from top 15% income counties - Within the higher SESs, BA+’s MI rates are similar to or better than Top 15%’s MI rates in both study periods: this is reasonable since we previously concluded that BA+ provides a stronger segmentation than Top 15%.
MI has slowed down in the short term, but materially positive MI can still be observed for the higher SESs - Rate of MI has generally slowed down in the short term (2011-2016) compared to the long term (2000-2016); all population subsets were affected by this slowdown, but to different extents:
- The higher SESs had slower MI in 2011-2016 compared to previous study periods, but the MI rates observed during this recent study period were still mostly positive and significant, with the exception of attained age group 35-44.
- The lower SESs had negative MI rates in 2011-2016, with the exception of attained age group 75-84.
Key Takeaways
When actuaries develop long term MI assumptions for life insurance using the U.S. general population data, it is important to account for the SES impact. Over the past few years, speed of mortality improvement has slowed amongst the general population. Despite the slowdown, materially positive MI can still be observed for the higher SESsC. Actuaries may want to take this into consideration before setting MI assumptions for life insurance portfolios.
While this paper discussed two methods to segment the U.S. population mortality data by SES: by education; and by county income, the next article of this series will combine our findings from the first two articles and discuss the impact of SESs on various causes of death.
Going forward, Munich Re’s Biometric Research team will continue to monitor emerging mortality improvement trends. We anticipate an update to our MI analysis in 2020 using the latest CDC data which will include 2018 population mortality. We look forward to sharing these additional insights as experience emerges.

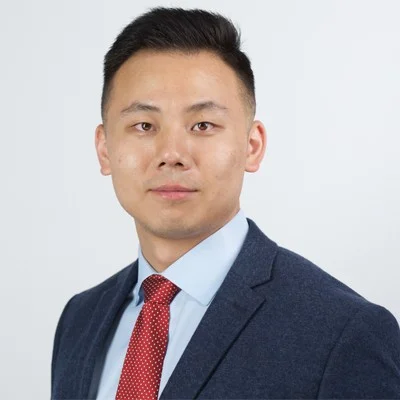