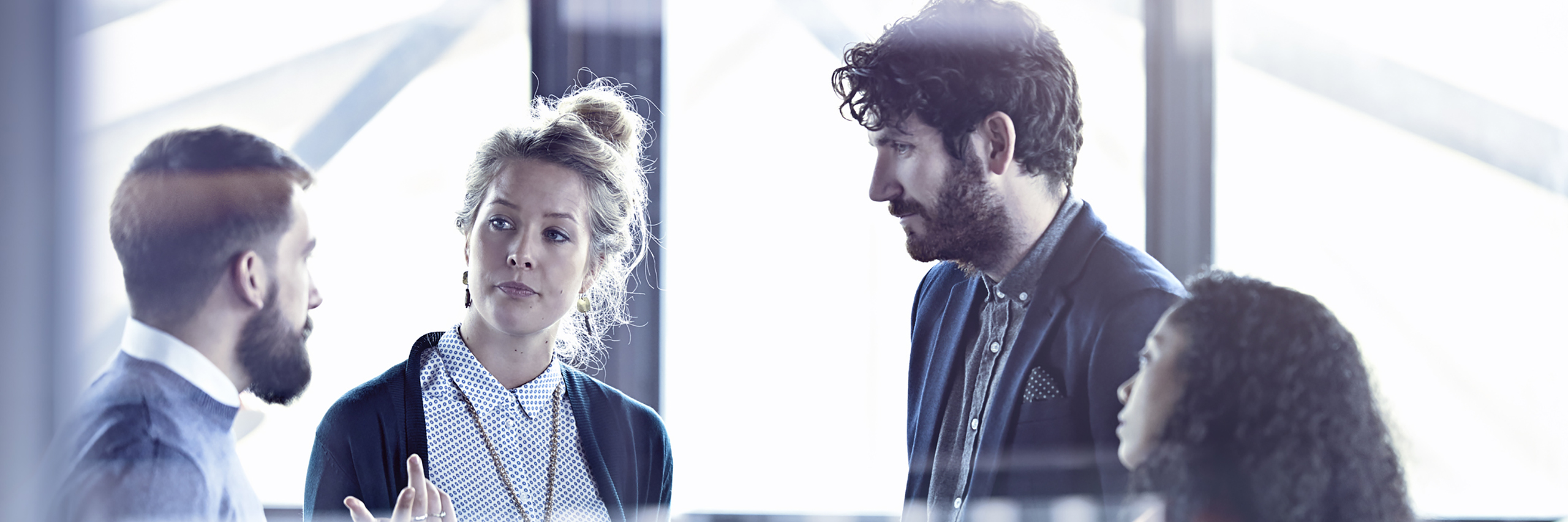
Analyzing Individual Life insurance Mortality Trends - Cause of Death Impact
properties.trackTitle
properties.trackSubtitle
Munich Re’s North American biometric research team continually monitors industry experience (mortality, morbidity, lapses). We hope to stimulate discussion with our business partners and across the insurance industry by sharing our key findings. We believe these discussions will lead to a better understanding of emerging experience and its importance in assessing underlying risk.
This paper is the first in a series that takes a deep dive into analyzing U.S. population mortality trends by cause of death (COD), and how these trends could be translated to the individual life insurance population. The analysis shown in this paper will address the following key question:
Is the recent slowdown of mortality improvement observed amongst the U.S. population also happening in the individual life insurance population, and what are the implications for the future?
In January 2019, the Society of Actuaries (SOA) published, “U.S. Population Mortality Observations – Updated with 2017 Experience”1, which analyzed U.S. population mortality trends using data published by the Centers for Disease Control and Prevention’s (CDC) Wide-ranging Online Data for Epidemiologic Research (WONDER) database, released in December 2017.
Amongst many observations made in this SOA report, we highlight the following:
- “The overall mortality rate (both genders) from all causes of death increased 0.4% in 2017, which followed a 0.6% decrease in 2016. Mortality has not improved cumulatively (both genders) since 2012.”
- “Heart disease and cancer stand alone as the only two CODs that showed improvement (excluding CODs within the Other category) over 1999-2017, 2012-2017 and 2016-2017. But heart disease improvement has slowed notably in more recent periods. Conversely, cancer improvement accelerated.”
- “The combination of higher cancer improvement combined with slowing heart disease improvement and generally deteriorating mortality for most other CODs resulted in no mortality improvement for all CODs from 2012-2017.”
The rest of this paper will discuss how, and to what extent, these trends relate to mortality improvement in the insurance population.
Causes of death: population vs. insurance
The SOA report highlighted the observation that mortality trends are not homogenous across different CODs. In order to understand the difference in mortality trends between the general population and insurance population, we need to identify the prevalence of each COD within the two populations.
Graph 1 shows the difference in COD distribution between the general population and Munich Re’s US individual life portfolio claims by count.
The general population COD distribution was derived from the SOA report appendices file1 by calculating the proportion of cause-specific mortality rates in each calendar year and taking an average for the 2007-2017 period.
The Munich Re’s experience is filtered for:
• Study period 2006Q3-2017Q2
• Duration 1-20
• Single life fully underwritten (No COLI/BOLI)
• Automatically reinsured (exclude facultative reinsurance)
• Excluded substandard and term conversion
Ideally, we would like to standardize the two data sources by taking into account differences in death distribution by age between Munich Re’s portfolio and the general population. This was not done in the current analysis due to data source limitations.

As shown in Graph 1, we observed that the Munich Re insurance portfolio causes of death skew significantly towards more cancer and less heart disease compared to general population, in particular for females. One possible explanation for this skewness is that life insurance underwriting is more effective at filtering out potential cardiovascular disease than cancer. Traditional underwriting methods such as blood tests and family history can be highly effective in determining the risk of heart disease, allowing high risk individuals to be excluded from the insurance pool or be charged a higher premium, which makes the product less attractive. In comparison, cancer is more difficult to predict through traditional insurance underwriting. This pronounced COD skewness toward cancer should be an important consideration when actuaries are setting mortality trend assumptions
for insurance portfolios.
Cause of death: face amount and gender impact
Diving further into Munich Re’s insurance portfolio COD distribution from the most recent experience (2012Q2-2017Q2), we further observed that:
· Policies with face amounts greater than $100,000 have a higher prevalence of cancer-related deaths in every age group compared to policies with under $100,000 face amount. This may be explained by:
o Many insurance companies’ underwriting guidelines historically required fluid tests to be conducted for policies with over $100,000 face amount, which effectively identified individuals with higher risk for heart disease.
o Higher face amount policies often belong to wealthier individuals with higher socio-economic status, which has an impact on the cause of death distribution.
· Females in the younger and middle ages (below age 75) have a higher prevalence of cancer-related death and lower prevalence of heart disease death compared to males. The relationship reverses at the older ages (age 75+). This may be explained by:
o Females on average develop their first heart attack seven to 10 years later than males2, possibly due to unhealthy habits more common amongst younger men, such as smoking, stress and a high-fat diet.
o Strong prevalence of breast cancer amongst the younger female population (below age 65)3.
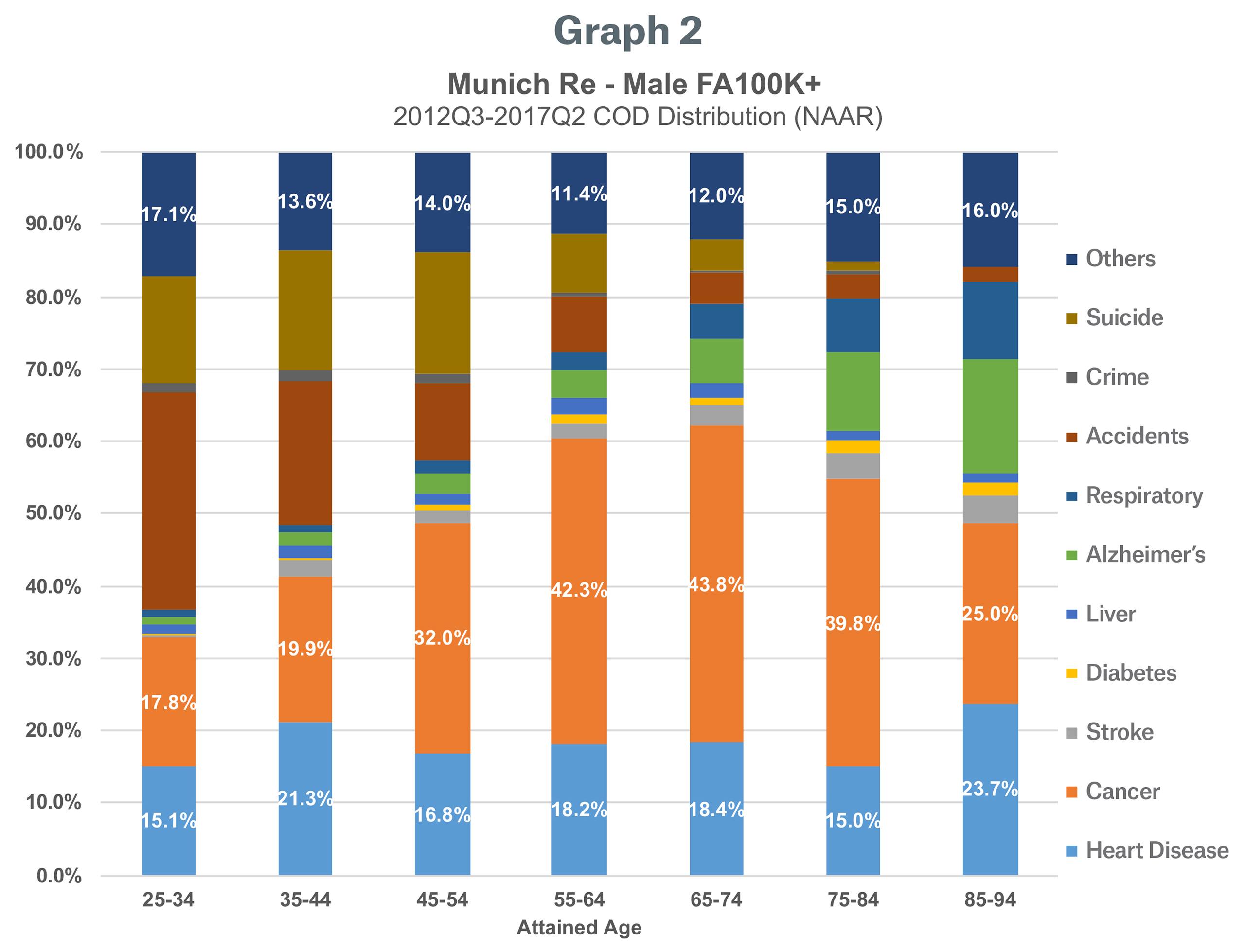
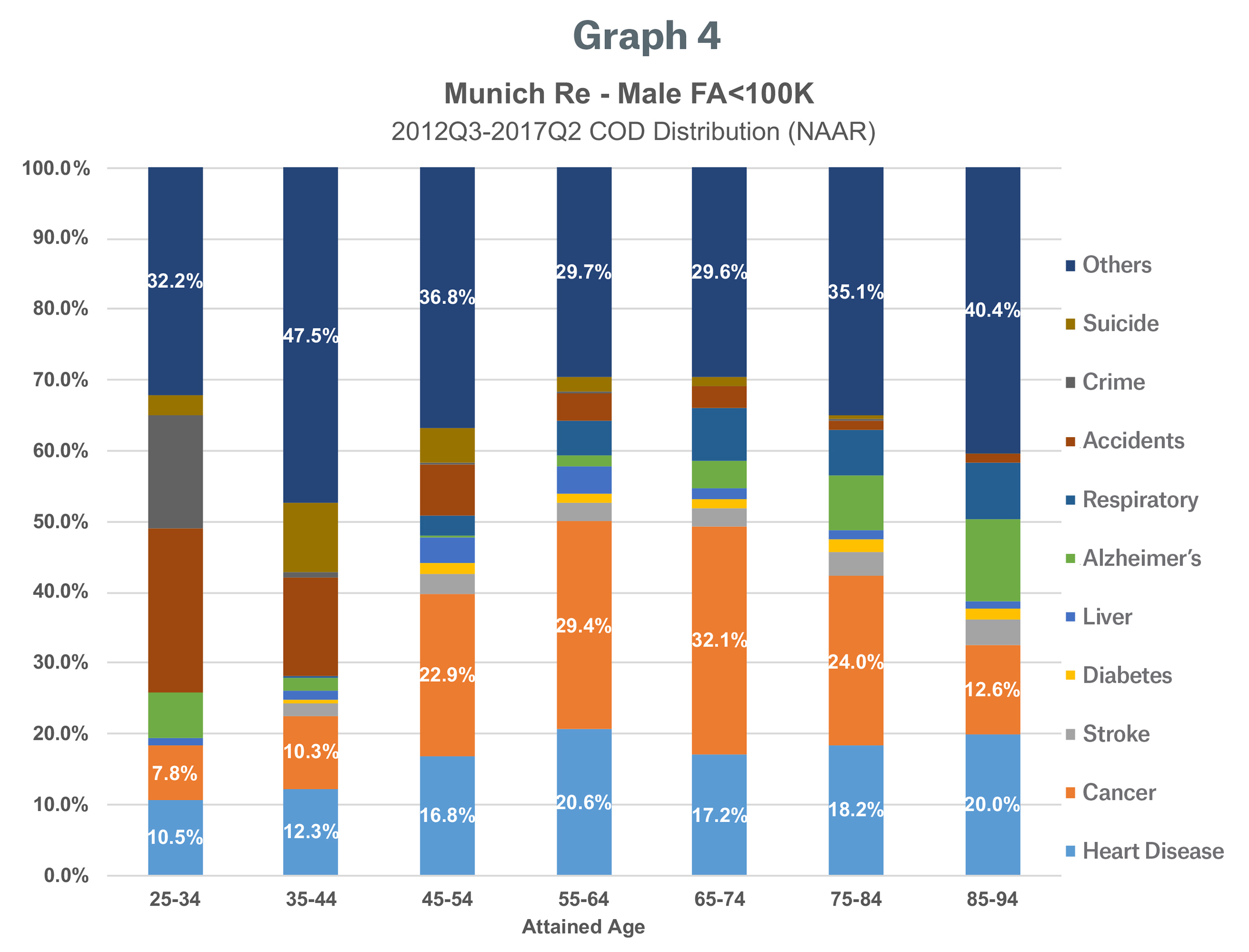


Mortality improvement implications
Given the difference in COD distribution between the general population and the
insurance population, it is likely that recent mortality trends may have impacted the two
groups differently.
The SOA paper pointed out that general population mortality improvement has
slowed and even showed disimprovement in recent years, largely caused by the slowdown in heart disease improvements, which overshadowed the acceleration in cancer
mortality improvements.
The most recent year of overall mortality deterioration (from 2016 to 2017) was -0.4%, which was mainly attributed to accidents and other COD, -0.2% and -0.3% respectively (page 6-7 of the SOA report). Opioid deaths4 were a key driver behind the 2017 deterioration in accidents. This negative improvement trend was changed to a slightly positive +0.1% when we excluded opioids from the calculation. Moreover, the +0.1% would increase further to +0.4% if population COD distribution was replaced with Munich Re’s insurance portfolio COD distribution.
The 0.8% gap (-0.4% vs +0.4%) between the initial population mortality improvement trend and the adjusted trend goes a long way towards explaining the differences in the rate of mortality changes between overall and insured populations from 2016 to 2017.
Conclusion
While the rate of mortality improvement has been slowing in the overall U.S. population,
the trend can look different for the life insurance population when COD prevalence is taken into consideration. Life insurance underwriting is known to be more effective at identifying certain risk factors than others, and each insurance portfolio’s COD distribution is impacted by the company’s underwriting program and target demographic. When actuaries review mortality improvement experience and set future mortality improvement assumptions,
it is important to take the COD prevalence into consideration.
As underwriting methodology evolves, many companies are starting to remove fluid tests and introduce new underwriting tools. It is important to understand the selective features of these underwriting tools, beyond measuring the protective value using a traditional A/E analysis. For example, even if a new underwriting method can fully offset the mortality A/E impact from removing fluid tests, it may still have a secondary impact on mortality improvement trends, because it may be identifying a different portfolio of individuals.
In the next paper in this series, we will further discuss segmenting the U.S. general population data by socio-economic status to derive improvement trends relevant to the insurance population. We believe that sharing our findings will lead to better understanding the key drivers behind emerged mortality improvement trends and hope to stimulate further discussions on how COD distribution can impact overall mortality trends in a life insurer’s block of business.
Recent Update: In a more recent report published by the SOA in June 2019,5 the mortality rate in the U.S. general population decreased by 1.6% from 2017 to 2018, with material decreases observed on all major CODs including heart disease, cancer, stroke and Alzheimer’s. We will continue to monitor this experience and conduct further research.

/Alex%20Yang.jpg/_jcr_content/renditions/original./Alex%20Yang.jpg)