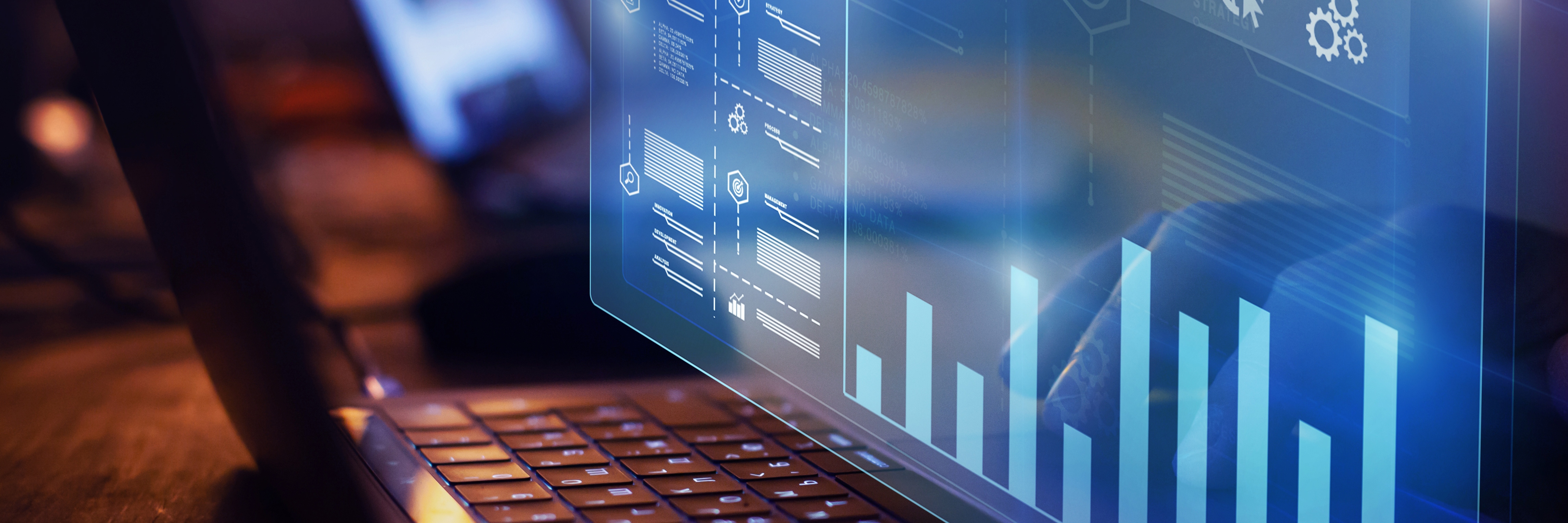
Taming the beast:
Challenges and considerations when implementing LLMs in insurance
properties.trackTitle
properties.trackSubtitle
February 2024
Our previous articles explored how large language models (LLMs) work and considered how LLMs could transform underwriting and claims management.
We now turn to the factors carriers must consider when investing in and implementing LLMs. Three critical areas are LLM accuracy, the developing regulatory landscape, and expected costs.
Assessing accuracy in LLMs
LLMs are black-box AI systems that use deep learning to understand and generate new text. Models like OpenAI’s ChatGPT and Google’s PaLM were created with general capabilities, such as “write a letter” or “write a poem.” Evaluation of these models is a challenge because LLMs are, by design, non-deterministic (hard to always predict the output) and language-based (hard to compare the output). Evaluation metrics such as ROUGE (Recall Oriented Understudy for Gisting Evaluation) and BLEU (Bi-Lingual Evaluation Understudy) are commonly used for accuracy testing. Another common approach is the use of benchmark datasets to compare model performance. These go by fun names such as GLUE, SuperGLUE, HELM, MMLU, and BIG-bench.
Benchmarks tend to measure engagement, task recognition, relevance, and specificity, which are all good measures for a general model. However, they are not specific to a given industry. Because LLMs frequently suffer from hallucinations, which are the generation of nonsensical or unrelated text, as well as brittleness, which refers to widely varying outputs resulting from small changes to inputs, a relevant benchmark and evaluation framework is vital to their adoption in situations where consistency and accuracy is a top priority.
As we apply LLMs to insurance use cases, new testing metrics and benchmarks will need to be developed. Due to the specific and precise nature of the insurance business, these frameworks must be rigorous and robust enough to give carriers a sense of comfort when deploying an LLM in a production setting. Generic frameworks have not yet reached this level of specificity in the insurance industry, hence the need for use case-specific frameworks for assessing accuracy.
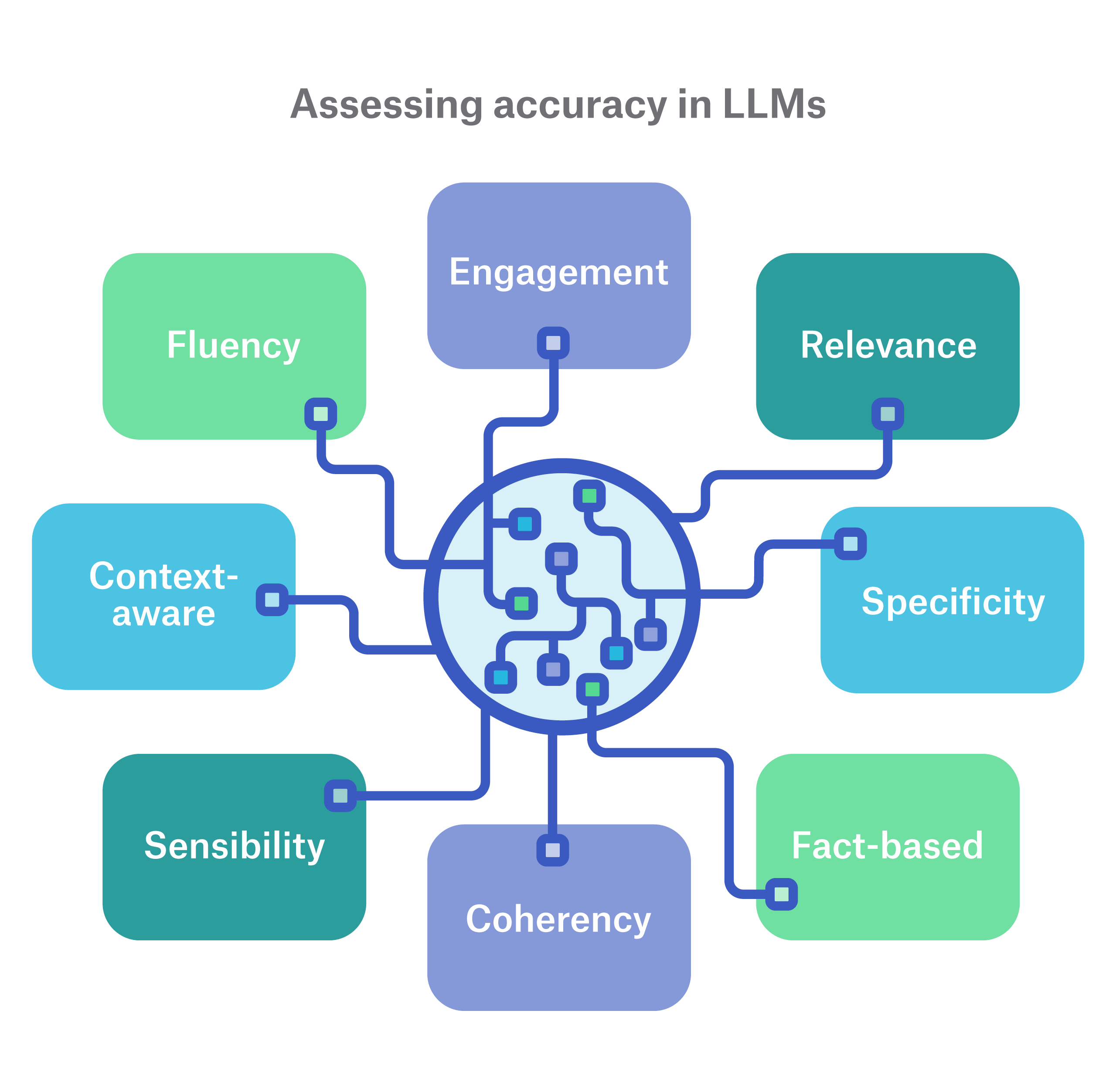
Legislative considerations
The groundbreaking nature of LLMs has raised genuine concern about the viability of these models as safe and reliable tools. Key considerations for legislative bodies include understanding how LLMs reach their outcomes (i.e., explainability) and establishing the guiding principles and specific rules by which they operate, with a focus on protecting the privacy and personal rights of individuals.
Insurers must reach a comfort level that LLMs work without bias, produce explainable outcomes, and won’t miss important information that can affect an outcome or decision. As a result, the LLMs we train and implement for insurance use cases require rigorous bias testing to ensure they meet any legislative guidelines ascribed to Automated Decision-Making systems (ADMs). Once complete, we can turn our attention to stress testing them to ensure they perform as intended; in other words, taming the beast.
We can expect and should welcome legislation regulating LLMs at this stage. Several jurisdictions are considering legislation aimed at limiting or controlling the use of generative AI (more specifically, LLMs) while maintaining consumer rights to information and objection.
In Canada, Federal Bill C-27 seeks to modernize and replace its data privacy legislation, the Personal Information Protection and Electronic Documents Act (PIPEDA), and introduce the Artificial Intelligence and Data Act (AIDA). In Quebec, Law 25 focuses on ensuring the transparency and accountability of Automated Decision Making systems that use personal information.
In the U.S., the National Institute of Standards and Technology (NIST) has held public-private sector discussions to develop national standards for creating “reliable, robust, and trustworthy AI systems,” and at least 25 states introduced bills to examine and establish AI standards during the 2023 legislative session.1
Any legislative framework developed will be general, and insurers will need to outline more specific practices for our industry that focus on accountability, compliance, transparency, and security. It will be important for the industry – reinsurers, carriers, and insurance regulatory bodies – to come together and ultimately create practical legislative frameworks for LLMs in insurance.
Cost considerations
There’s little doubt that the insurance industry will eventually reach a comfort level with LLM viability, accuracy, and adherence to regulation. However, the question of cost does remain. As a critical factor in operationalizing LLM technology, carriers need to evaluate the expected costs for hosting, inference (i.e., generating text outputs), training, and, of course, skilled resources. We can think of these cost areas as an inverted triangle, with hosting at the bottom since this cost is generally definitive and training at the top because of its wide cost variance.
Hosting and inferencing costs are usually combined and depend on the model size – the number of parameters is a good proxy– and how frequently the model is used for inferencing. Recall that these models are large, and their sheer size means they require multiple GPUs for hosting and inference. Multi-GPU cloud configurations result in higher costs than are typically required for normal servers. For example, a seven-billion parameter model could cost upwards of USD 30,000 per year for hosting, while a 40-billion parameter model could cost upwards of USD 180,000. Size and usage are significant factors when considering the costs.
Training is a totally different ball game. Training costs vary greatly, from hundreds or perhaps thousands of dollars for parameter-efficient fine-tuning (PEFT) to millions for full fine-tuning. Most organizations perform some level of PEFT where only a subset of the original weights (or a small number of extra weights) are fine-tuned, significantly lowering costs. Although not specifically classed as training (where model weights are modified), retrieval augmented generation (RAG) is a cost-effective technique allowing LLMs to operate only on information stored within special databases called vector databases. This approach is widely used to significantly lower “training” costs within an order of hundreds or perhaps thousands of dollars.
Resource costs, such as those needed to create data sets for training, are usually overlooked. Regardless of the technique employed, these models need data. Curating large data sets for training will take time and resources. This will add additional resource costs that should not be ignored.
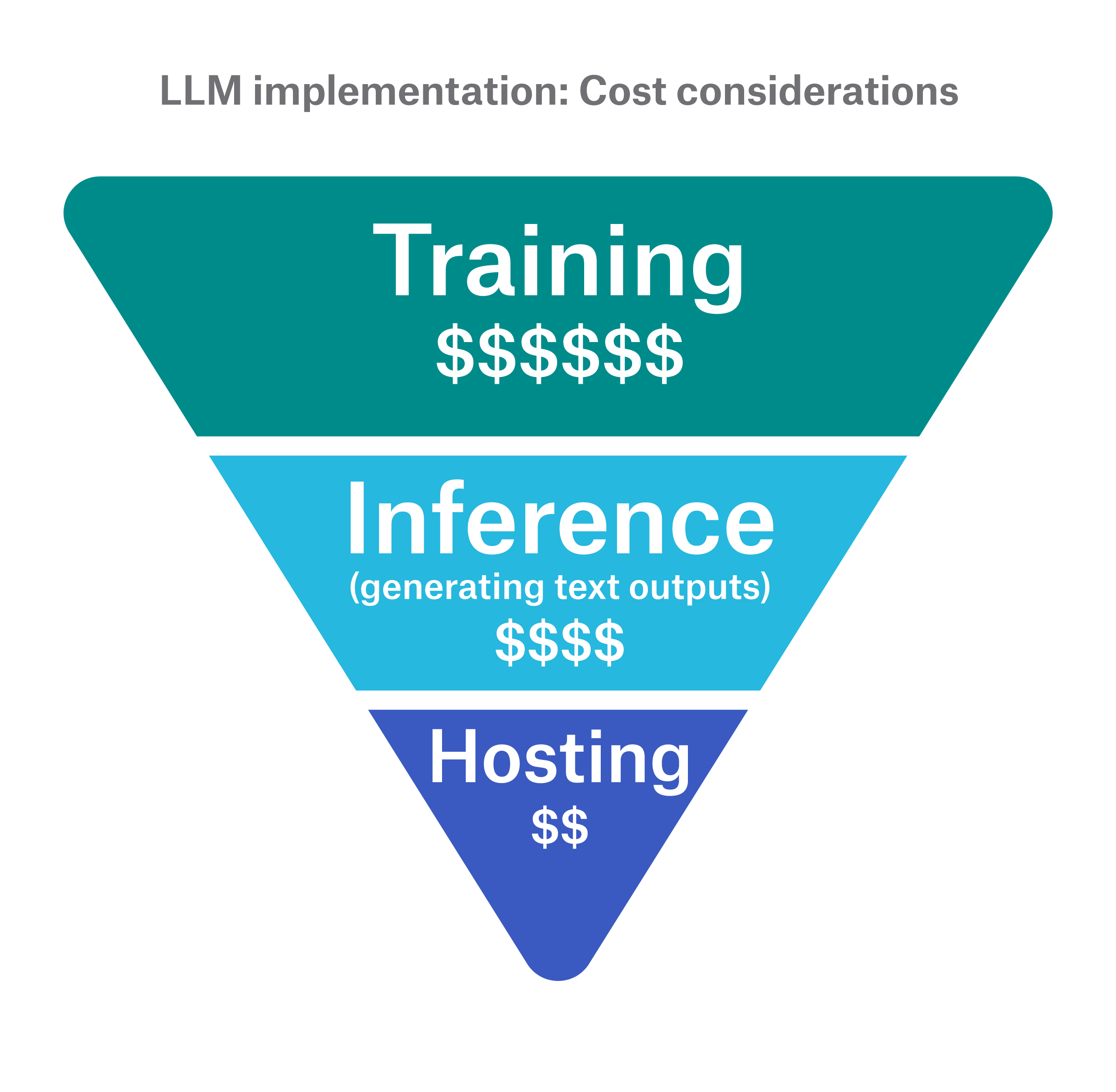
A look forward
LLMs are transformative in their ability to harness difficult-to-reach information efficiently. They can synthesize unstructured data from different sources and personify that information, potentially providing insurers with a new and efficient way to interact with their most valuable data. Carriers with valuable, domain-specific, proprietary unstructured data (e.g., medical underwriting data) could create a competitive advantage by harnessing previously inaccessible information from such data.
The insurance use cases are many. In this article series, we touched on two areas - underwriting and claims – where LLM adoption could be particularly game-changing.
Yet, with any promise comes reality. LLMs can be costly to train and operate. However, workarounds do exist to lower costs, and the underlying technologies, as well as the evaluation frameworks, are improving. In addition, legislation focused on protecting privacy and personal rights is a priority for governments. These are excellent building blocks to ensure a future where the adoption of this technology does not outpace its ability to be controlled.
As a result, there is a huge upside for LLMs in insurance. Working together as an industry to establish rigid principles governing the adoption of this technology will help ensure that we tame the beast and implement LLMs in the most advantageous ways possible.
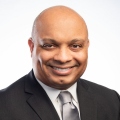