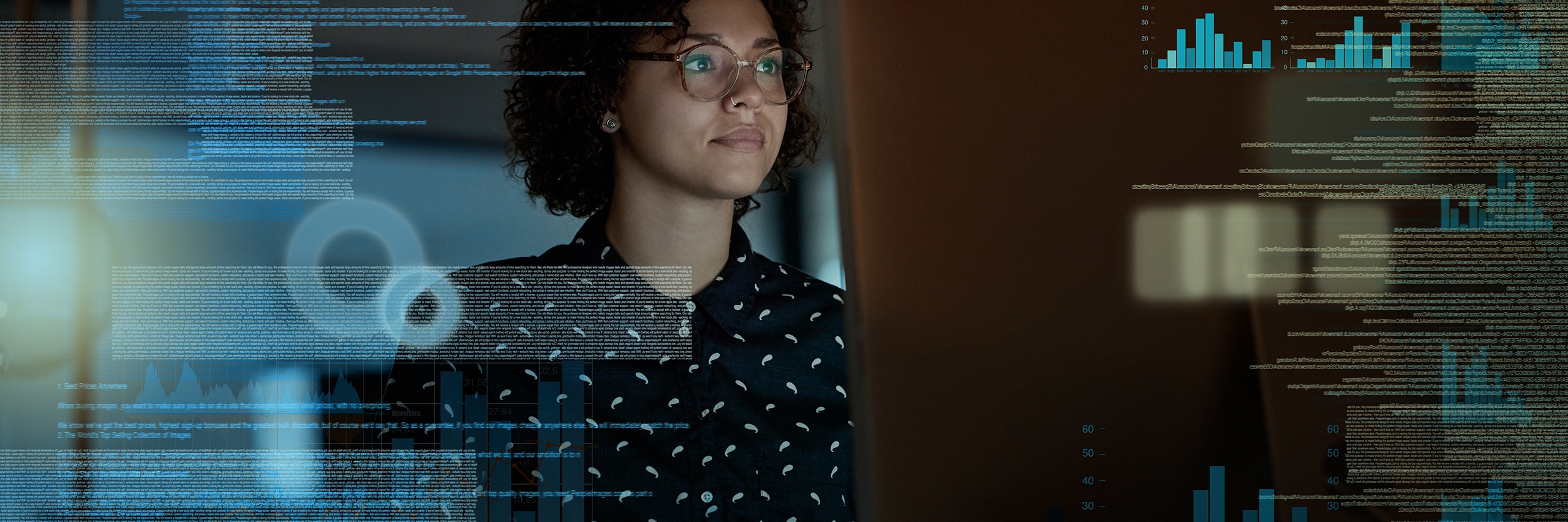
Sleep and Mortality
Analyzing the effectiveness of daily sleep duration in stratifying mortality risk
properties.trackTitle
properties.trackSubtitle
June 2020
For over 50 years, doctors and scientists have recognized “the critical importance of sleep to good health and life.”1 Though initial studies focused on sleep deprivation, the full body of research now shows adverse health impacts for both overly short and long sleep. Atypical sleep duration is associated with adverse medical conditions, such as cardiovascular disease, obesity and physiological stress, along with resultant higher mortality; age, gender and socioeconomic status mediates these relationships.2
We analyzed the effectiveness of daily sleep duration in stratifying the mortality risk profile of a U.S. insured population simulated from National Center for Health Statistics (NCHS) survey data.
Munich Re analyzed the effectiveness of daily sleep duration in stratifying the mortality risk profile of a U.S. insured population simulated from National Center for Health Statistics survey data.
Data and Extrapolation to Life Insurance
The data analyzed was derived from the National Health and Nutrition Examination Survey (NHANES) conducted by NCHS, a program of studies designed to assess the health and nutritional status of adults and children in the United States. The survey examines a nationally representative sample of about 5,000 persons each year, and sampling weights are provided to ensure the weighted results are indicative of the country as a whole.3
Data was limited to survey years when sleep information was collected, which spans 2005 to 2014. Mortality information, which NCHS sources from the Social Security Administration and Centers for Medicare and Medicaid Services, is available through December 31, 2015, with an average follow-up time of six years in the final sample. Fields available in the data include:
- Demographic information including age, gender and household income
- Medical examination and laboratory data including BMI, blood pressure and cholesterol
- Self-disclosed smoking status and history of cancer, stroke, and diabetes
- Self-disclosed typical hours of sleep on weekdays/workdays
- Vital status of each individual and, if relevant, the date at which death was observed and high-level underlying cause of death categorization
Using this data, Munich Re simulated an insurable population by selecting lives with:
- Age 18 to 84
- Household income greater than $20,000
- No history of cardiovascular disorder
- No history of cancer (other than non-melanoma skin cancer)
- BMI within insurable limits (16 – 48)
The insurable population consists of 19,037 lives and 800 deaths. Each life is weighted by the interview weights provided by NHANES so that the final dataset is roughly equivalent to an insured population drawn from the U.S. population at the midpoint of the survey period.3
Key Findings
- Self-reported hours of weekday sleep effectively stratifies mortality risk.
- Individuals with atypically short or long daily sleep duration have elevated mortality, controlling for gender, age, and smoking status.
- Relative mortality for long sleep (10 hours or more) is approximately 2-3 times greater than for 7 hours of sleep, which is the population median and generally recommended amount of sleep.
- The adverse impact of long sleep duration on mortality is greater for older individuals than younger individuals, whereas the adverse impact of short sleep duration is greater for younger individuals than older individuals.
Classic Actuarial Methodology
We performed a classical actuarial actual-to-expected (A/E) mortality analysis on the simulated insurable population dataset. The expected mortality basis shown in the following plots was taken from the 2015 Valuation Basic Table (VBT) primary select and ultimate Age Last Birthday (ALB) tables split by age, gender and smoker status. 2015 VBT actual-to-expected ratios are presented relative to a 100% fit for the entire simulated population.
To confirm the reasonability of the filters applied to identify insurable individuals in the survey dataset, we also applied the expected mortality basis taken from the Human Mortality Database (HMD) U.S. Life Tables, which represents U.S. population mortality split by age, gender and calendar year.4 The overall A/E using the HMD basis is 74%, consistent with expectations that an insurable population is healthier than the general population.
Overall Results
Figure 1a shows that the bulk of individuals sleep six-eight hours each night, with a median of 7 hours, which aligns with the generally recommended amount of sleep for an adult. However, there is still a significant population (15%+) with short or long daily sleep.
Figure 1b demonstrates that hours of weekday sleep stratify mortality risk, with both the “short sleepers” and “long sleepers” showing significantly higher mortality, making a characteristic U-shape in the one-way A/E plot. Short sleep duration (under 6 hours a night) and long sleep duration (more than 8 hours) is a risk factor for weight gain, hypertension, cardiovascular disease, and Type 2 diabetes.5
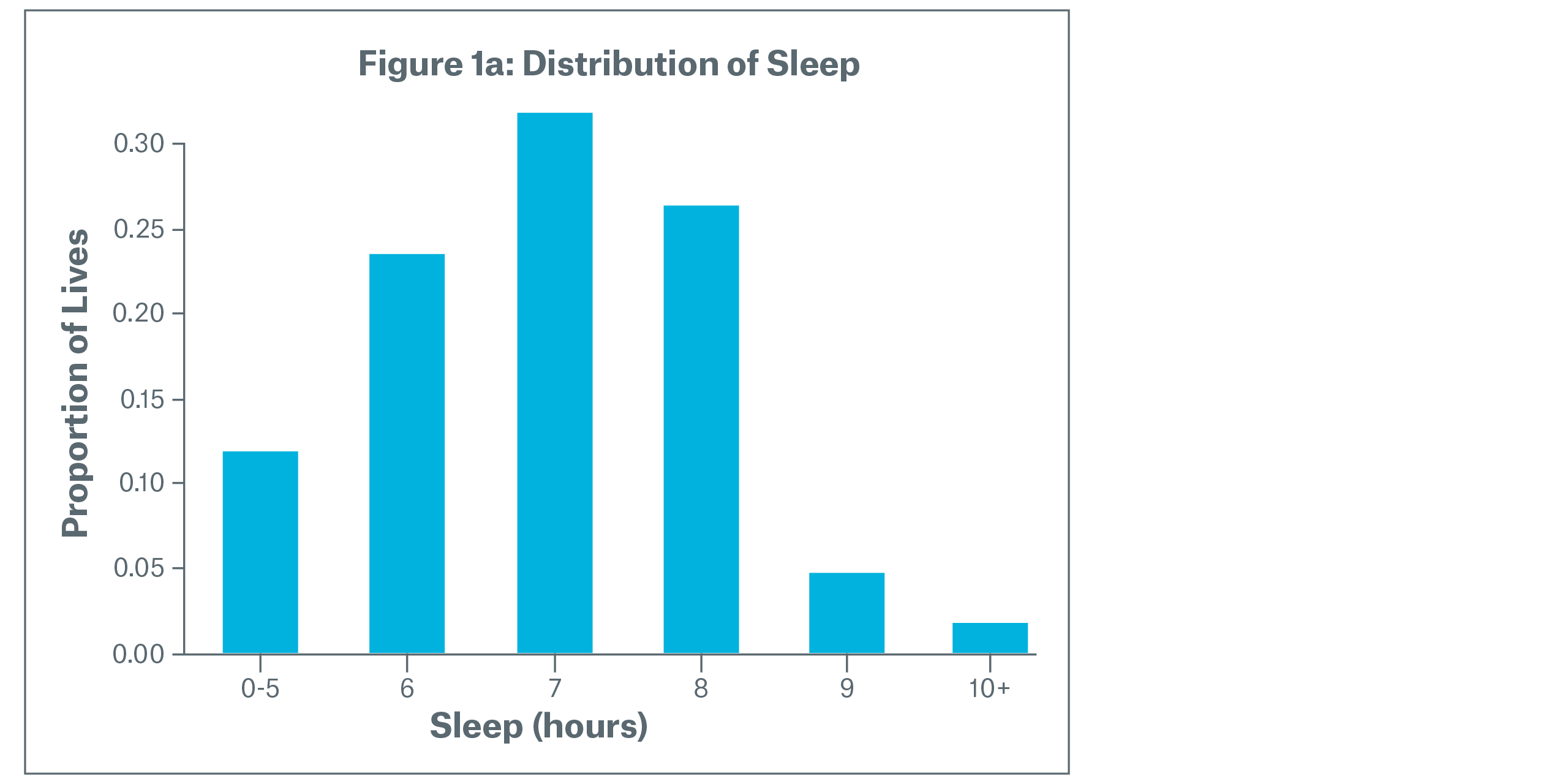
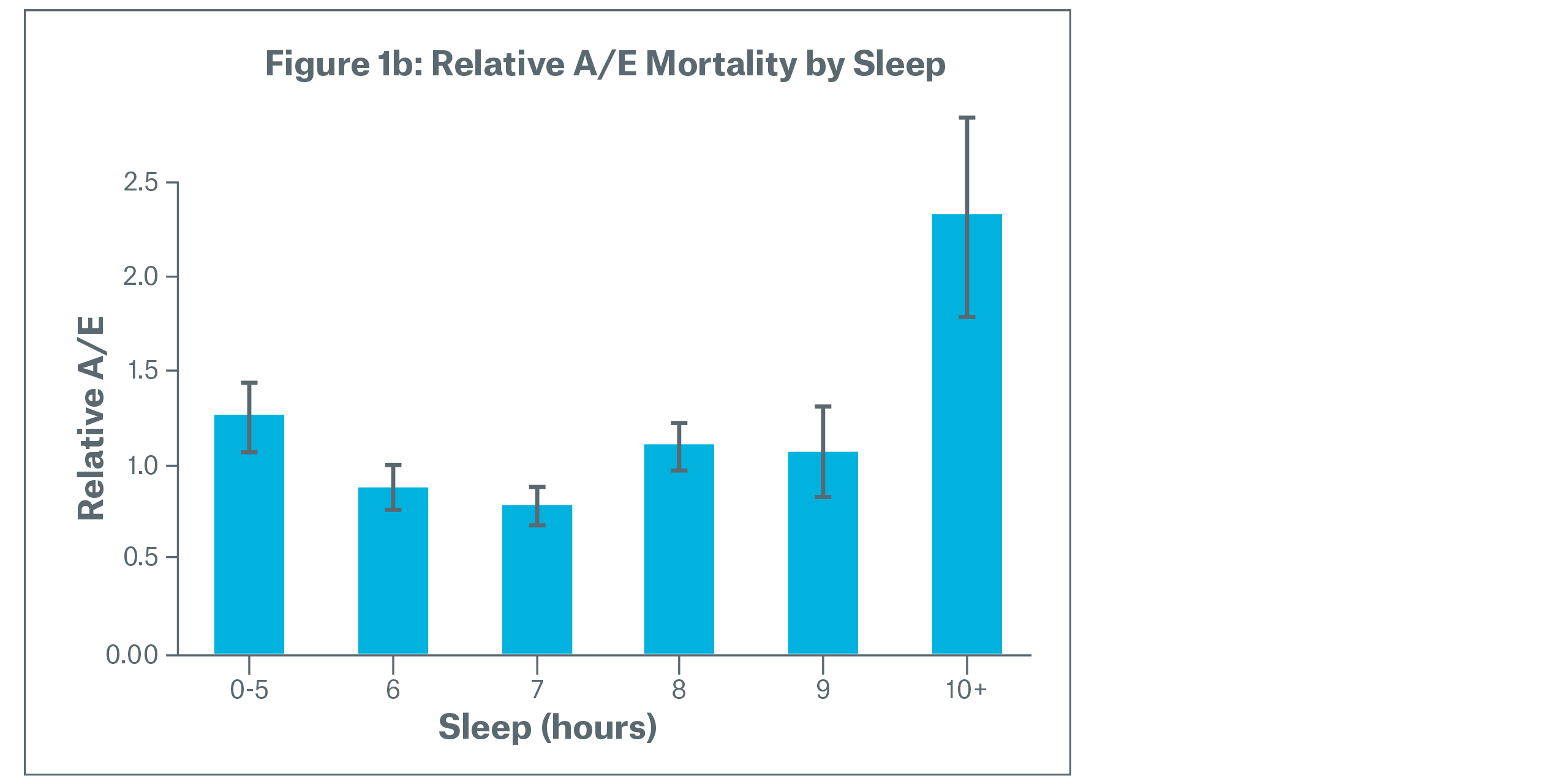
By Age
Although patterns of sleep vary throughout a person’s lifetime, reparative sleep needs remain relatively constant throughout adulthood. Older individuals tend to report more sleep interruptions or difficulty falling asleep, related to changes such as shift in circadian rhythms, increase in sleep disorders or other illnesses, and use of medications that disturb sleep.6 Figure 2a shows the distribution of daily sleep distribution by age, with individuals under the age of 30 dropped due to lack of credible death data for these younger ages. The oldest age range, 70-84, experiences longer sleep duration than younger ages, which is slightly counterintuitive to the expectation that older individuals would have more medical conditions that disturb sleep. It is possible that the elimination of cardiovascular disorders and cancer in the insurable population simulation may be partially responsible for this reversal.
The A/E bars in Figure 2b illustrate that short sleep duration can be particularly harmful for ages 30-49, though there is also somewhat limited death data for these younger ages. Excessive hours of sleep is harmful across all age groups compared to the baseline of seven hours.
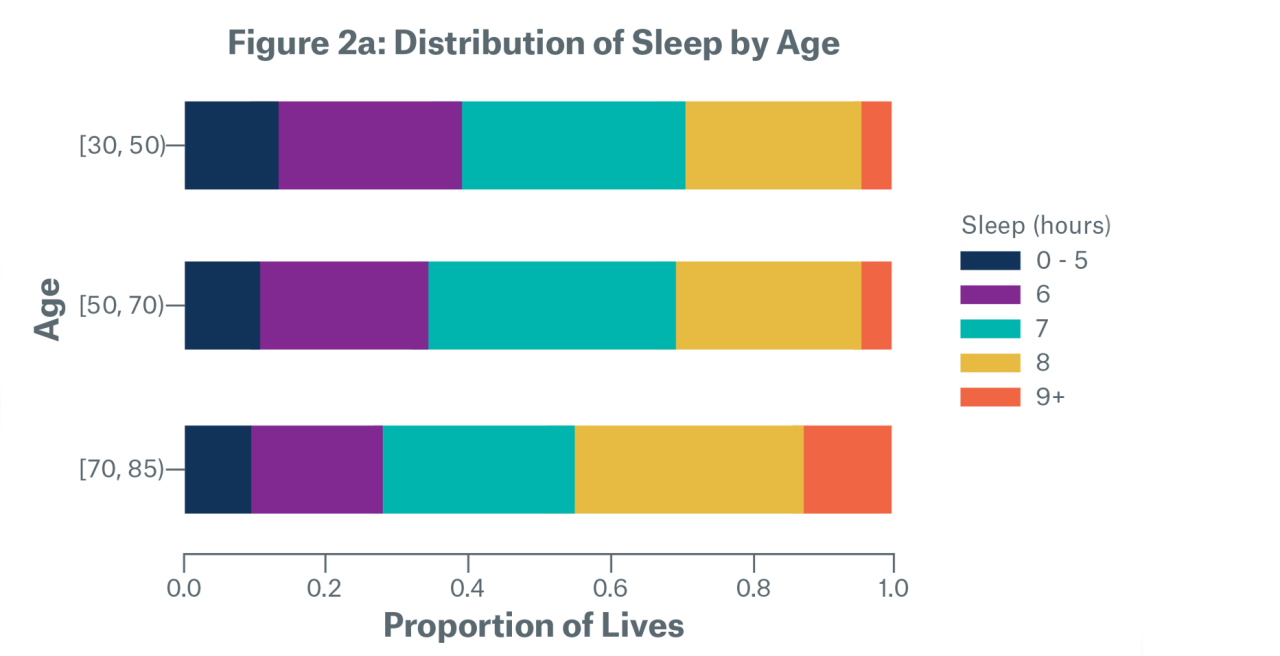
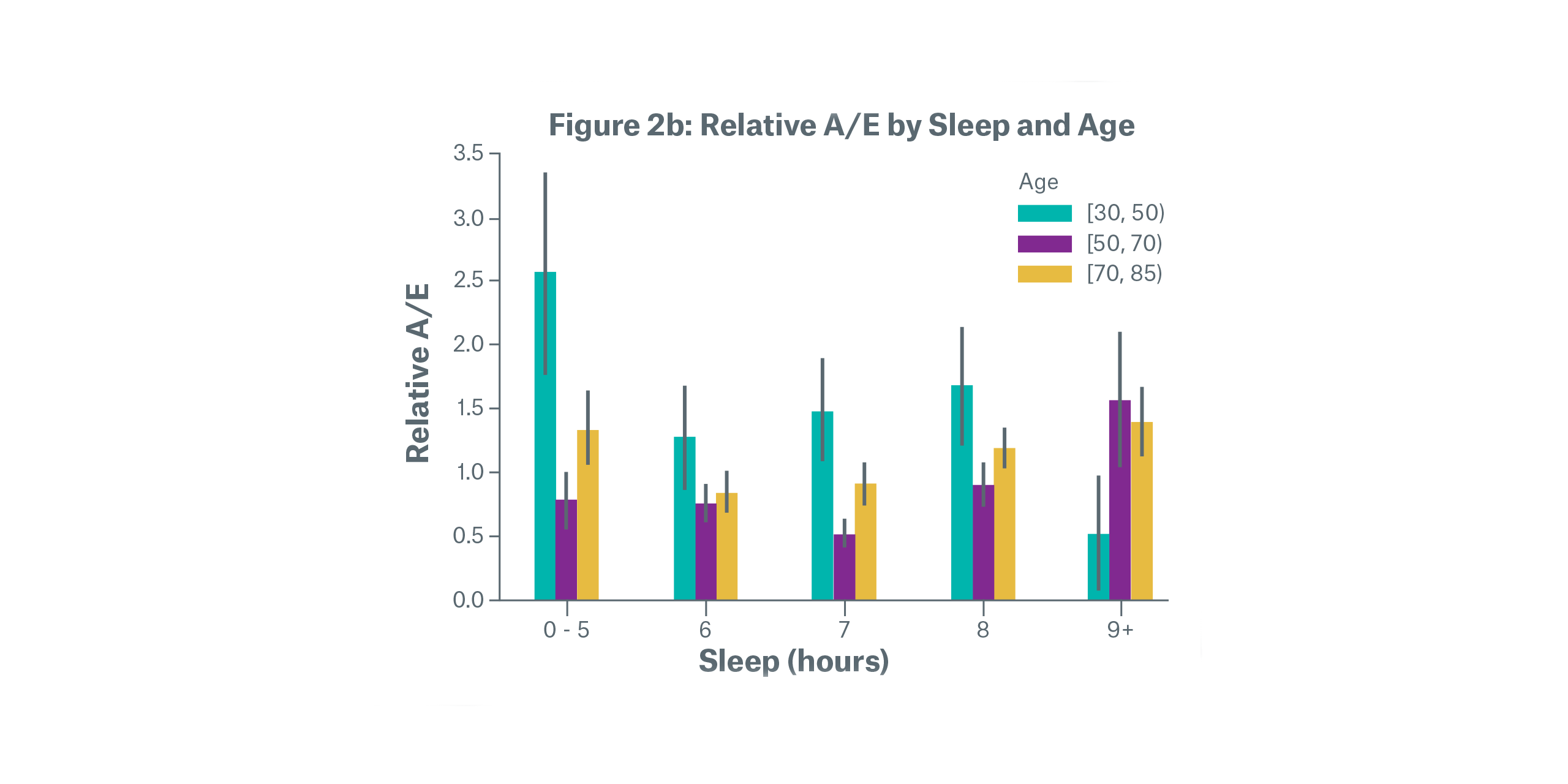
By Gender
Female median sleep is slightly greater than male (Figure 3a), while Figure 3b shows that mortality segmentation is present for both males and females in the familiar U-shape, though the curve is generally steeper for males, especially at shorter sleep durations.
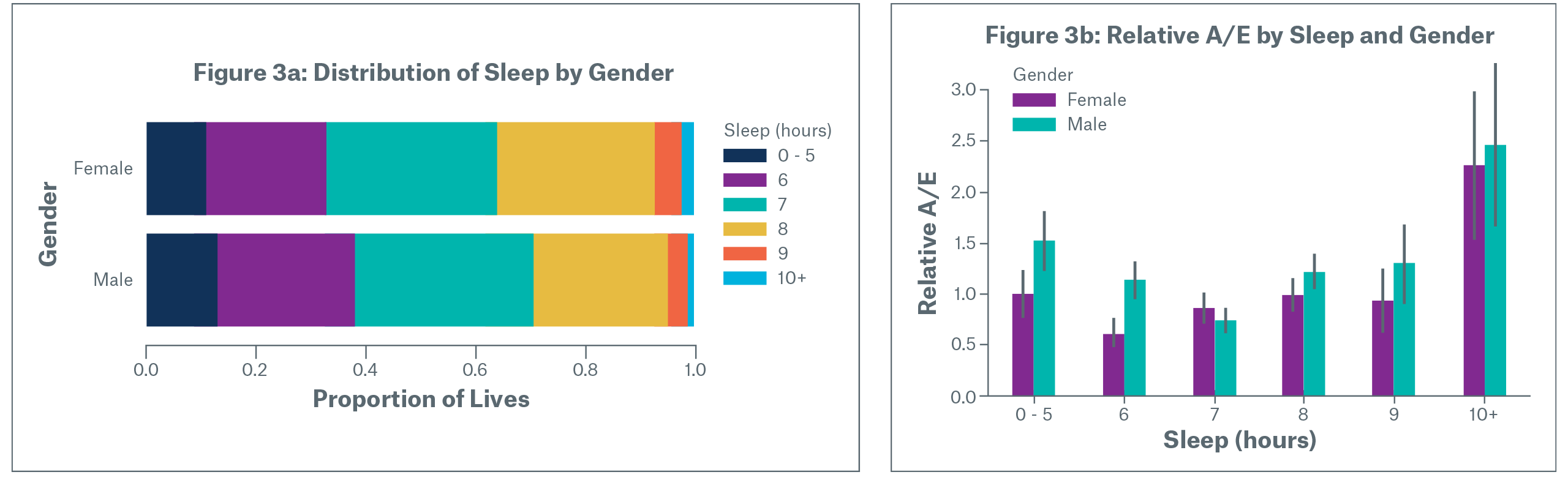
By BMI
As BMI increases, sleep duration decreases slightly, shown in Figure 4a. This pattern is in line with research pointing to fewer hours of daily sleep being associated with weight gain.2 Consistent with other splits of the data, both atypically short and long duration remain harmful across BMI groupings.
Note that BMI 16-24.9 represents underweight to healthy individuals, with very few in the underweight category; BMI 25-29.9 represents overweight individuals, and BMI 30+ represents obese individuals.
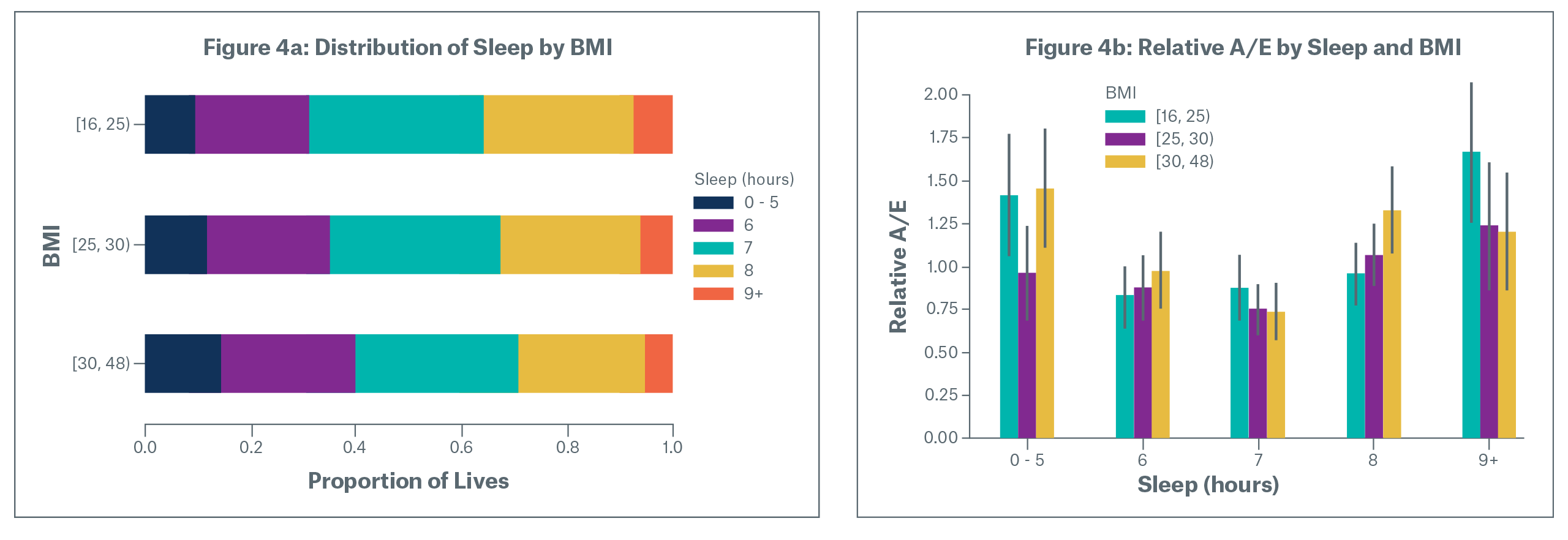
Cause of Death
As discussed above, abnormal sleep duration is a risk factor for cardiometabolic risks, including heart disease, so we expected to see an association between actual causes of death related to heart disease and self-reported sleep hours. High level cause of death groupings are provided in the NHANES survey results, summarized as the three cause of death categories shown in Figure 5. Figure 5 demonstrates that individuals self-reporting either short or long sleep duration have an increased proportion of deaths attributable to heart disease or stroke.
One caveat is that “voluntary” short sleep (such as an individual spending less time in bed) versus short sleep due to an underlying disorder (such as insomnia or obstructive sleep apnea) cannot be distinguished in self-reported sleep, and these two scenarios likely exhibit different pathways for the impact of sleep duration on health and mortality.5 However, if the ultimate outcome is higher risk in either scenario, then sleep duration is still an appropriate segregator even if the exact mechanism is unknown. Relationships between sleep and mortality are also mediated by socioeconomic status so trends may be attenuated when indicators of socioeconomic status are included in the analysis.
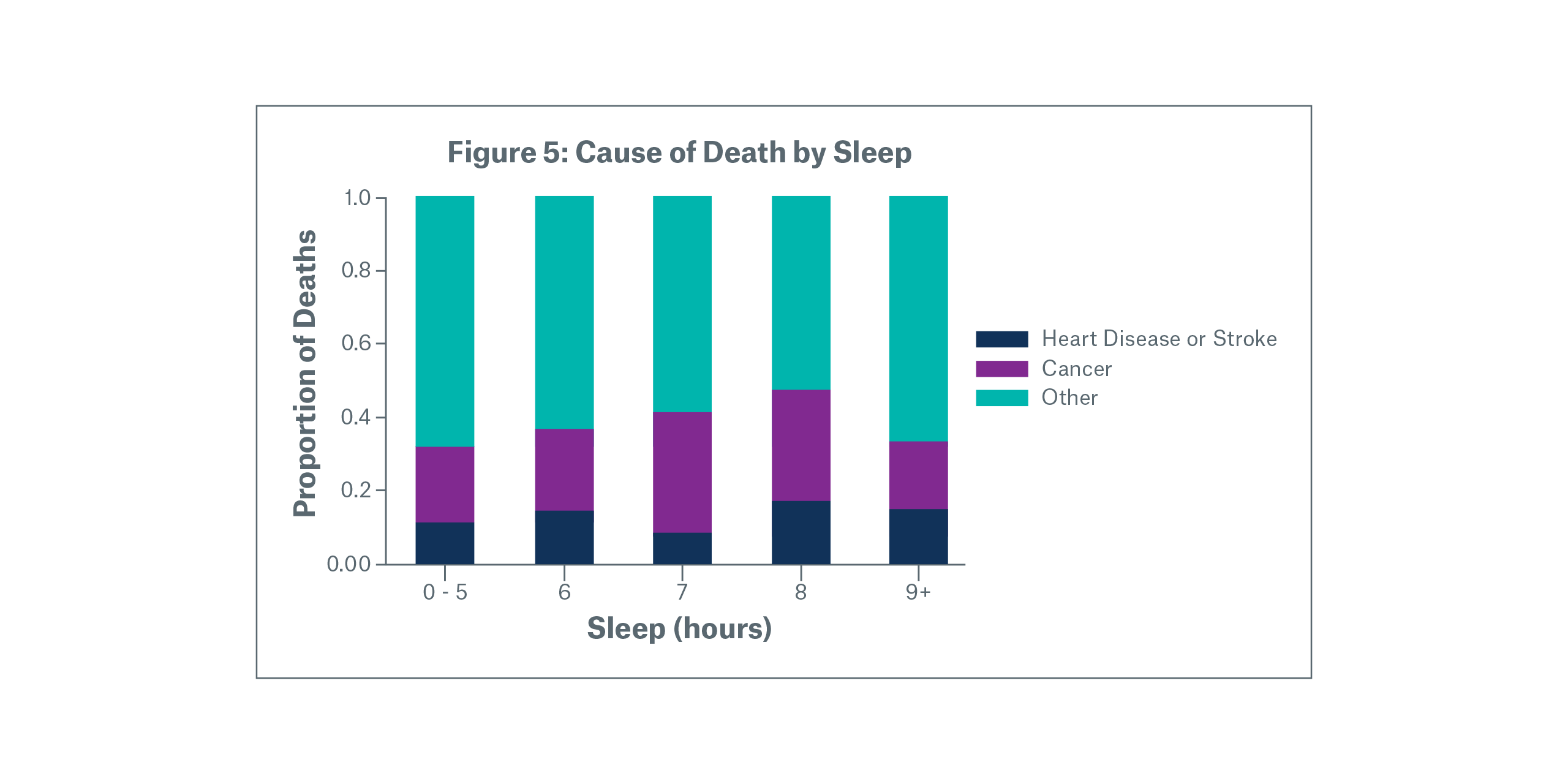
Modeling
Complementing the classical actuarial mortality analysis, Munich Re applied predictive modeling techniques to evaluate the extent to which sleep predicts mortality. Modeling can reveal additional insights beyond one and two-ways analyses when multiple variables are related to both each other and the outcome of interest. Modeling can also smooth noise and help trends become more apparent.
Poisson regression was used to model the actual to expected deaths explored in the previous section. This model isolates the impact of sleep on mortality controlling for age, gender, smoking status, and duration. The target of the model is actual death count, and the covariates are hours of weekday sleep and the interaction of sleep with age, with the log of expected deaths appearing as an offset (covariate with fixed coefficient of 1).
Figure 6 shows a comparison of A/E as predicted by the model compared to the original, traditional one-way approach. The model output aligns nicely with the classic approach with a clear smoothing effect. The model itself allows us to explore the interaction effect of age and hours of sleep. In Figure 7, we use the model to predict A/E across a range of sleep values for specific age and gender combinations. The A/Es were then normalized so that predicted A/E at 7 hours of sleep is 100% across all ages, making it easier to compare mortality trends across age bands.
Figure 7 shows that the predicted A/E curve increases more steeply for older individuals (e.g., the 75-year-old) than younger individuals as daily sleep duration increases. This indicates that long sleep has a greater influence on mortality for older individuals, an insight that was less apparent in Figure 2b’s two-way analysis due to noise associated with subdividing the data.
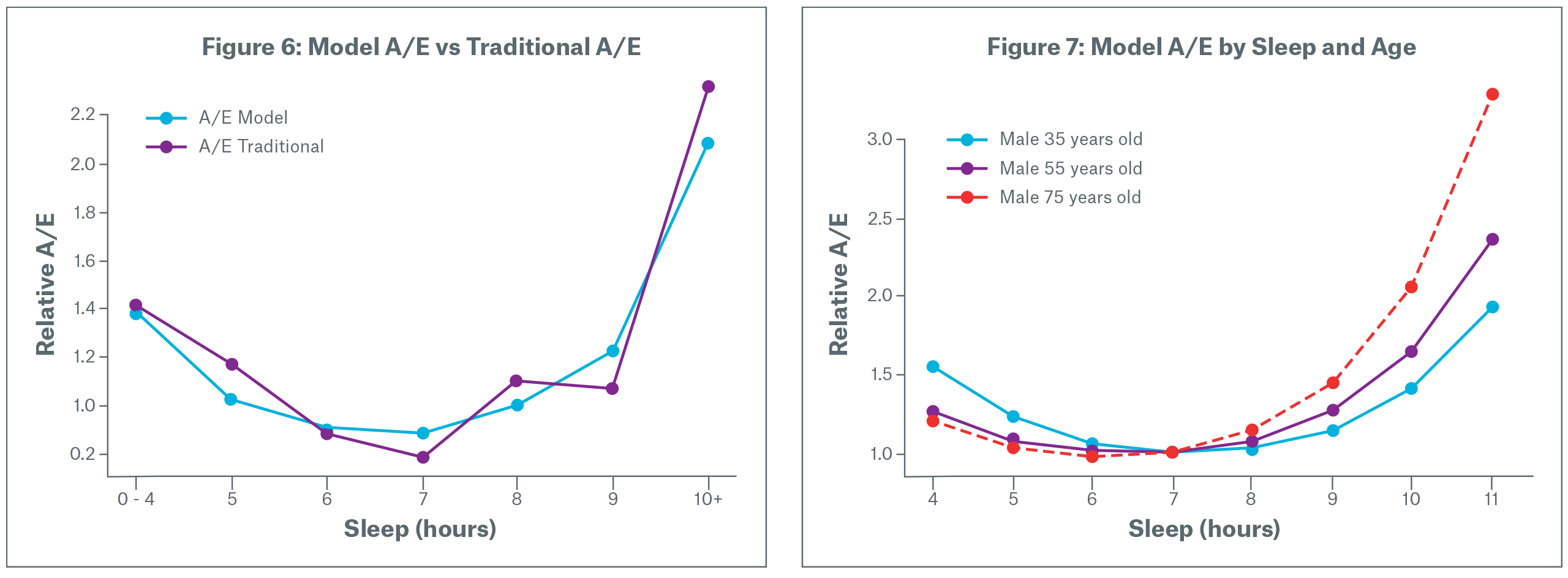
Conclusion
Based on our analyses, we conclude there is strong evidence that sleep can effectively segment mortality risk even after controlling for age, gender, and smoking status. High risk individuals may be identified by abnormally low or high self-reported hours of daily sleep.
The potential for applicants to falsely report better sleep results also must be considered; measured sleep duration data would be more desirable to obtain as opposed to self-reported sleep statistics. Although it is not feasible to verify sleep through labs as carriers do for other common sources of applicant misrepresentation (smoking behavior or BMI), many consumer wearables are capable of tracking sleep (or at least proxying hours of sleep), and this data could be collected from applicants. Common sleep tracking options include Fitbit devices, select Garmin fitness devices, and third-party apps compatible with Apple Watches.
A credible pool of life insurance applicants with historical sleep data (preferably captured through wearables) and mortality outcomes would most accurately measure the impact of incorporating sleep in life insurance risk assessment. A 2015 survey reported that 28% of individuals use a health app to track how long they sleep.7 A more informal survey of CNET readers in 2019 found that 30% of respondents tracked their sleep, with 73% of those affirmative responders using wearable devices.8 Users who track sleep are also skewed younger and more affluent, aligning with the target demographic for many products sold through accelerated underwriting programs, where sleep duration could act as an additional data point in the risk assessment process.
Insurers interested in adopting a sleep assessment or wearables program should begin with a pilot to appropriately collect information, assess consumer adoption rates and better understand the sleep characteristics of their customers. The pilot serves as a baseline analysis to support a more comprehensive customer engagement or risk assessment program. Munich Re Life US’ Integrated Analytics team has expertise in program development, measurement and monitoring – and can provide innovative solutions to carriers interested in exploring how to incorporate sleep duration in the life insurance process.
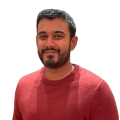
/Julia%20Druce.jpg/_jcr_content/renditions/original./Julia%20Druce.jpg)
1 Shepard, J. W., Jr, Buysse, D. J., Chesson, A. L., Jr, Dement, W. C., Goldberg, R., Guilleminault, C., Harris, C. D., Iber, C., Mignot, E., Mitler, M. M., Moore, K. E., Phillips, B. A., Quan, S. F., Rosenberg, R. S., Roth, T., Schmidt, H. S., Silber, M. H., Walsh, J. K., & White, D. P. (2005). History of the development of sleep medicine in the United States. Journal of clinical sleep medicine : JCSM : official publication of the American Academy of Sleep Medicine, 1(1), 61–82. Retrieved from https://www.ncbi.nlm.nih.gov/pmc/articles/PMC2413168/ 2 Grandner MA, H. L. (2010). Mortality associated with short sleep duration: The evidence, the possible mechanisms, and the future. Sleep Med Rev, 191–203. Retrieved from https://pubmed.ncbi.nlm.nih.gov/19932976/ 3 CDC/National Center for Health Statistics. (2017, September 15). About the National Health and Nutrition Examination Survey. Retrieved from CDC/National Center for Health Statistics: https://www.cdc.gov/nchs/nhanes/about_nhanes.htm 4 Human Mortality Database. Retrieved from University of California, Berkeley (USA), and Max Planck Institute for Demographic Research (Germany): www.mortality.org 5Knutson, K. (2010, October). Sleep duration and cardiometabolic risk: A review of the epidemiologic evidence. Best Practice & Research Clinical Endocrinology & Metabolism, 24(5), 731-743. Retrieved from https://doi.org/10.1016/j.beem.2010.07.001 6 National Sleep Foundation. (2009). Aging and Sleep. Retrieved from SleepFoundation: https://www.sleepfoundation.org/articles/aging-and-sleep 7 NYU Langone Health / NYU School of Medicine. (2018, January 17). Who uses phone apps to track sleep habits? Mostly the healthy and wealthy in US. Retrieved from ScienceDaily: www.sciencedaily.com/releases/2018/01/180117085748.htm 8 Turrentine, L. (2019, 12 17). Not tracking sleep? It's official: You're missing out. Retrieved from CNET: https://www.cnet.com/news/sleep-tracking-study-shows-health-improvements-and-more/