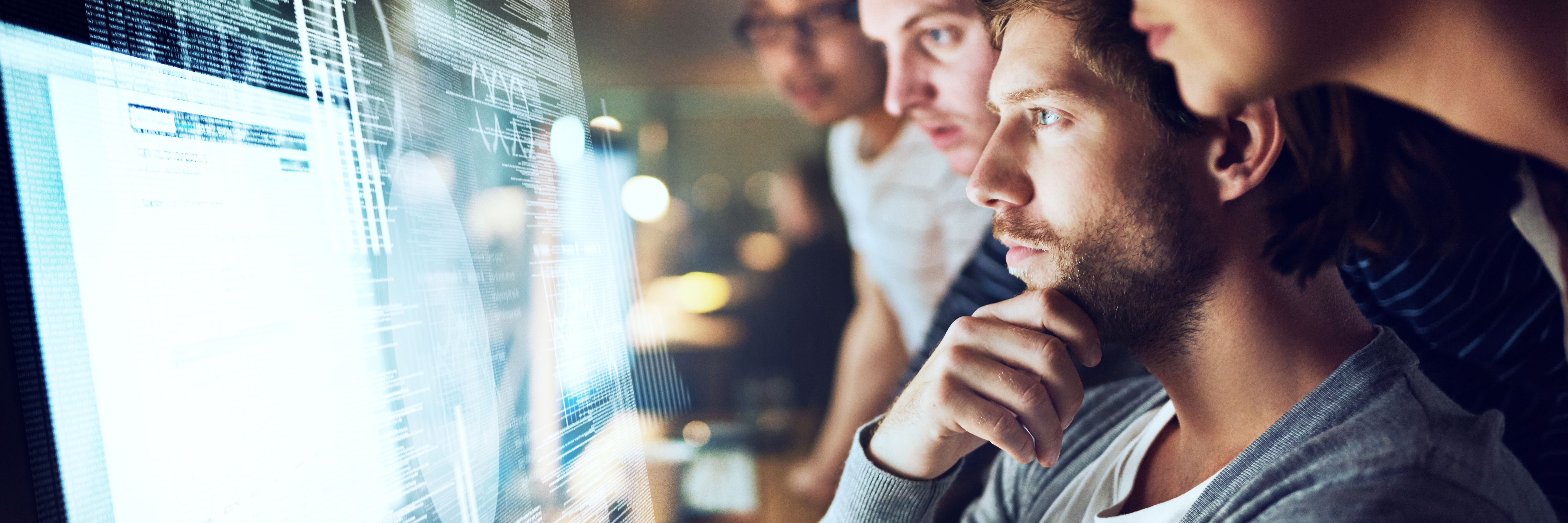
Creating an AI Strategy from the Ground Up
properties.trackTitle
properties.trackSubtitle
In the early 1990s, artificial intelligence (AI) was deemed a solution looking for a problem. Fast forward thirty years and AI is a mainstream discipline impacting almost all aspects of our lives. For insurers today, ignoring the potential that AI offers puts them at risk of falling behind their competitors. Yet many struggle to establish a solid AI strategy.
Crucial to fulfilling the promise of AI is creating a clear link between business value and AI capabilities. After identifying the business value, many insurers still find it challenging to move AI projects beyond the proof of concept stage and into production. CIOs, IT experts, and business leaders face many questions like: Where do we even start? What are our specific objectives? What talent do we need? And should we buy or build?
In the insurance industry, the AI capabilities most commonly deployed include predictive analytics, classification, and natural language processing. These techniques span the intersecting and sometimes confusing domains of Machine Learning and Deep Learning. These can be used to solve specific problems, also known as use cases. Examples of use cases relevant to the insurance industry include targeting marketing to potential applicants, accelerating and automating underwriting, predicting misrepresentation, and managing risk exposure.
What is AI?
A simple definition is that it is an ecosystem of algorithms – or “recipes” – that accomplish tasks normally associated with human intelligence. AI includes many subfields – from machine learning to natural language processing – each of which is made up of its own specialized set of algorithms.
There are five main steps to building a successful AI strategy:
1. Create a clear corporate vision and set of objectives
The first step is to articulate a clear corporate or departmental vision and a corresponding set of business objectives that will leverage AI.
As an example, let’s say that ABC LifeCo’s vision is to increase its Critical Illness insurance sales. It plans to achieve this by using AI to target its offerings to the needs of individual customers. This requires establishing a set of specific objectives granular enough to be empirically measured. Examples might include an 80% customer response rate to marketing, a 50% increase in sales, and a 30% reduction in manual processes – measurable goals that together help advance the company’s vision.
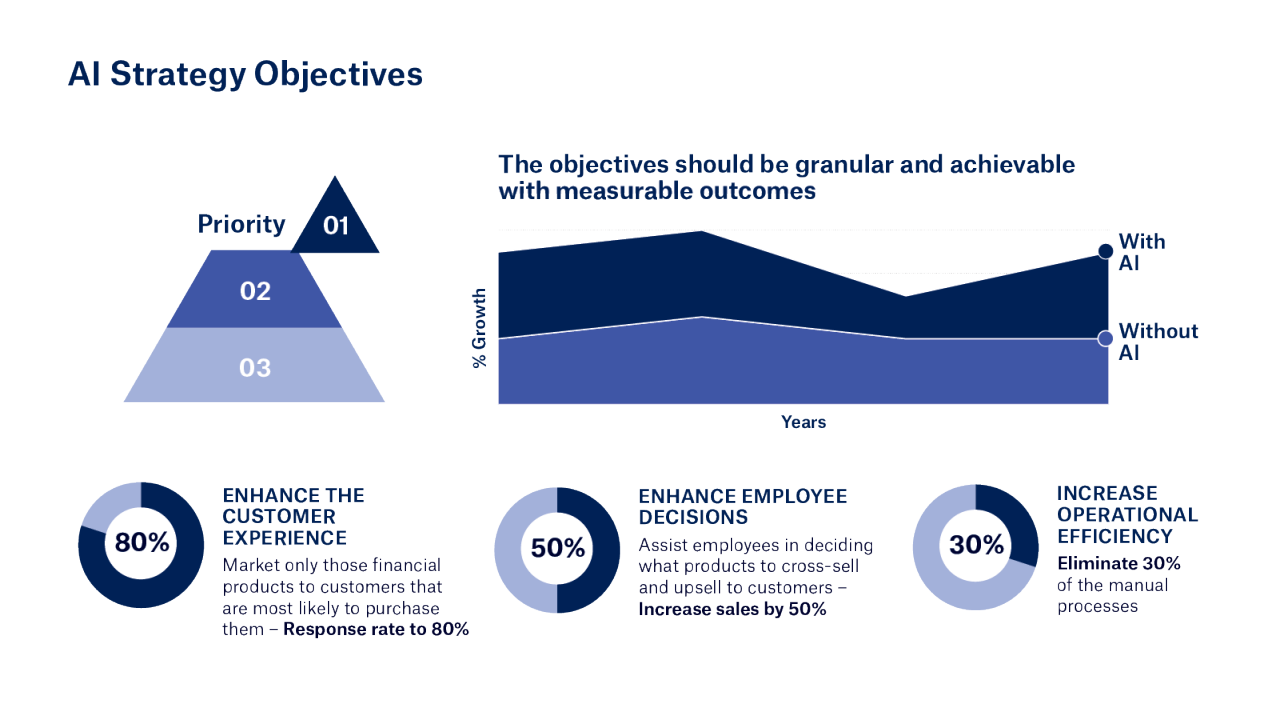
2. Define a set of use cases and assess the expected business benefit for each
The next step in launching a successful AI strategy is developing the specific use cases to which AI will be applied in order to achieve the desired outcomes. This is the stage at which the AI strategy really comes to life as leaders identify how the company will use AI and for what expected benefits.
Defining the use case starts with ideation, often through workshops with users, to get clarity on the specific business opportunity the company hopes to seize through the use of AI. Next, with the use case in mind, leaders should determine the expected business benefits of the use case and prototype a solution to prove those benefits, and assess the impact on current processes.
3. Identify the data and infrastructure necessary to support the use cases
With the use cases well defined, the next critical step is identifying the source and type of data needed to support them, as well as the technical infrastructure needed to collect, refine, analyze and model that data.
Companies may choose to build this infrastructure in-house, buy it all in the cloud or settle on a hybrid of the two. Given the maturity of available cloud services, most companies today are choosing a hybrid approach that enables some customization while also making it easy to get up and running quickly.
For example, Infrastructure as a Service (IaaS) allows companies to avoid hosting services on their premises, although they are still required to install and manage the AI platform. Platform as a Service (PaaS), on the other hand, is more expensive but gives companies full capabilities without the potential management headaches.
4. Acquire the appropriate talent and establish governance and a risk appetite
Building the right team, establishing the appropriate governance to implement and manage the AI strategy and ensuring the strategy aligns with the company’s risk appetite are also crucial to the development of a successful AI strategy.
Building the team
Traditional IT departments often need to bring in new talent to successfully implement AI pipelines. New hires most often include data engineers skilled in the preparation of data and systems engineers adept in implementing algorithms in the organization’s technical infrastructure. Also needed are experts in theory and modeling who are able to bring the state of the art in AI to the strategy or help the company develop new secret sauces. Some companies access this expertise through strategic partnerships with academic institutions rather than hiring in-house.
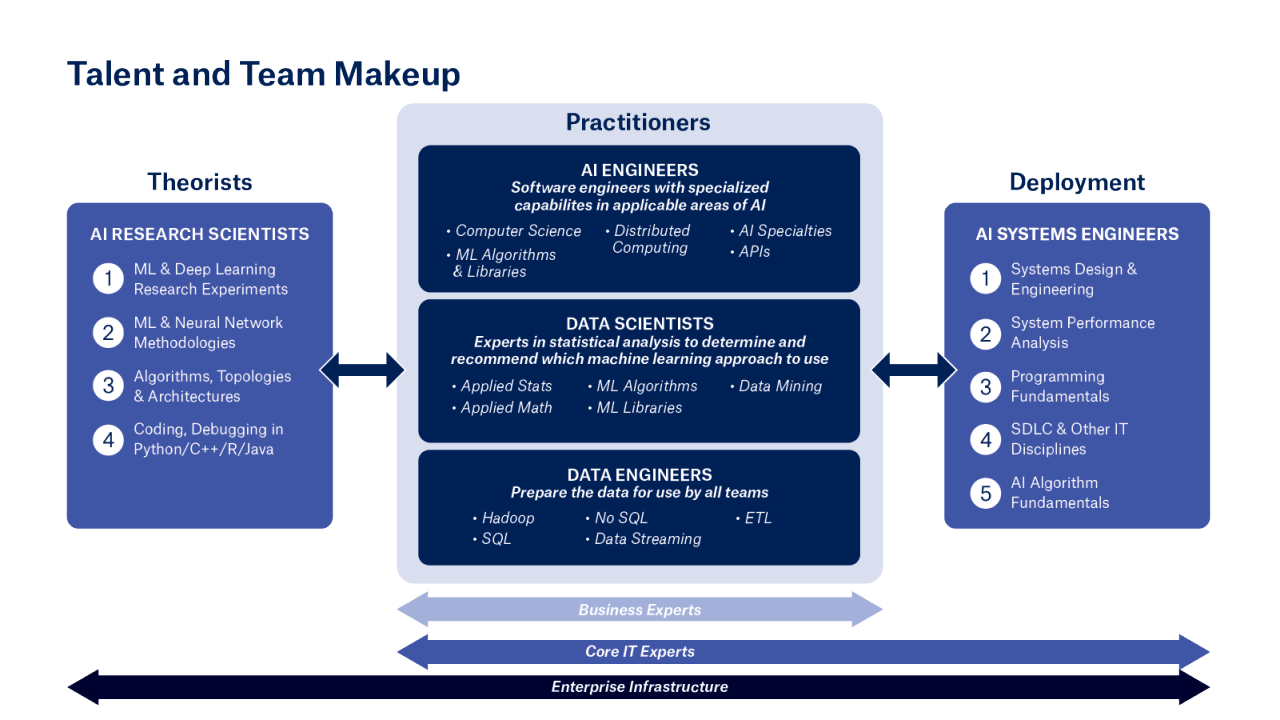
Governance and oversight
Effective governance is best achieved through a simple oversight structure headed by an Executive Steering Committee or ESC. The ESC is responsible for establishing the AI vision, principles, and guidelines. The ESC also oversees the Design Authority and various other working committees. The Design Authority should assess and approve any new tools, algorithms, and services added to the ecosystem, while the working committees focus on the day-to-day – from use case and business case development to the measurement of AI system performance. Other committees may focus on the legality of the product, risk, bias, and compliance to with local and federal laws, as applicable. This setup of course must be tailored to the size and complexity of the company.
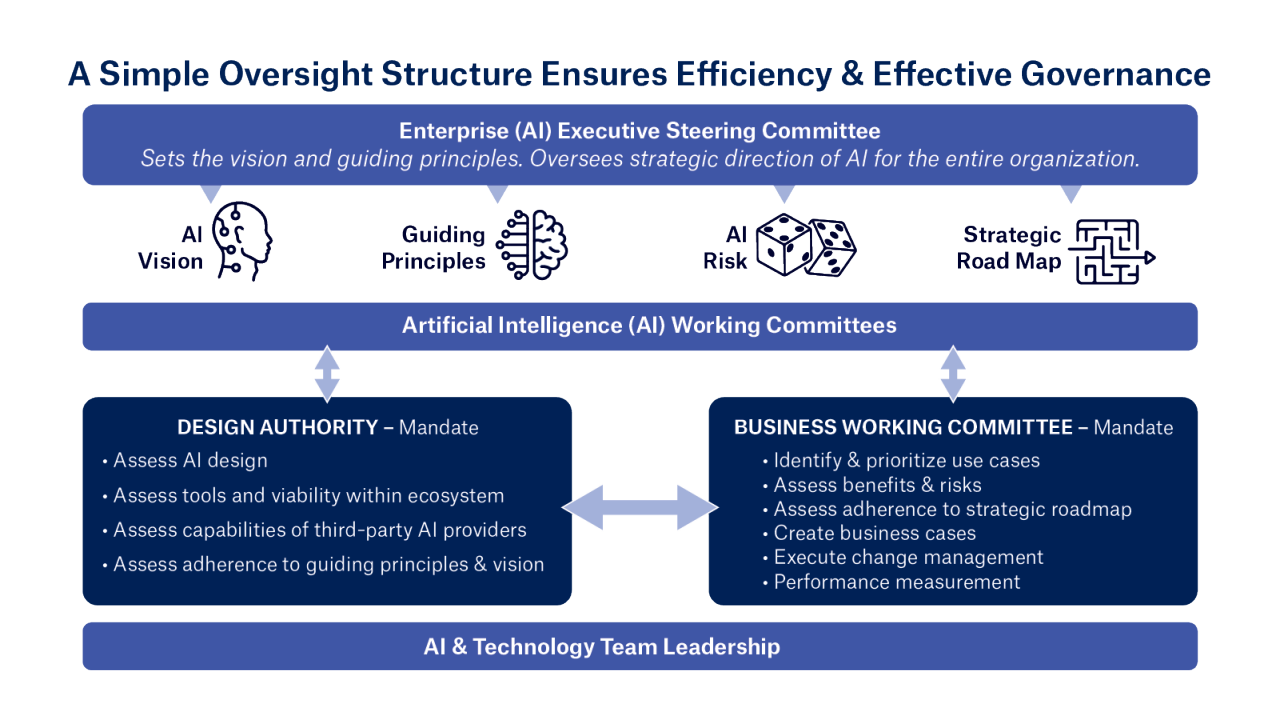
Assessing risk appetite
In building an AI strategy, companies must also consider their appetite for at least four types of associated risk:
- Legal risks related to the failure to adapt or comply with changing regulations on the use of AI;
- Model risks arising from incorrect or misused models;
- Reputational risk stemming from an AI-related activity that negatively impacts customers or stakeholders; and
- Operational risk related to the failure or disruption of internal processes.
5. Create a practical roadmap with immediate, medium- and long-term benefits
Finally, success is most likely when companies create a practical, incremental roadmap for implementation that allows them to build on previous successes. We recommend a 3-5 year roadmap that lays out priorities for the immediate, medium- and long-term as well as the sequence and duration of builds.
Below is a sample use case template for ABC LifeCo that reflects the steps discussed above. Note that the opportunity is clearly articulated, and the activity roadmap has simple steps that build upon previous capabilities and are designed to achieve the use case objectives. Also captured on the template are the AI techniques involved – essential information for the IT department – and the risk profile of the use case.
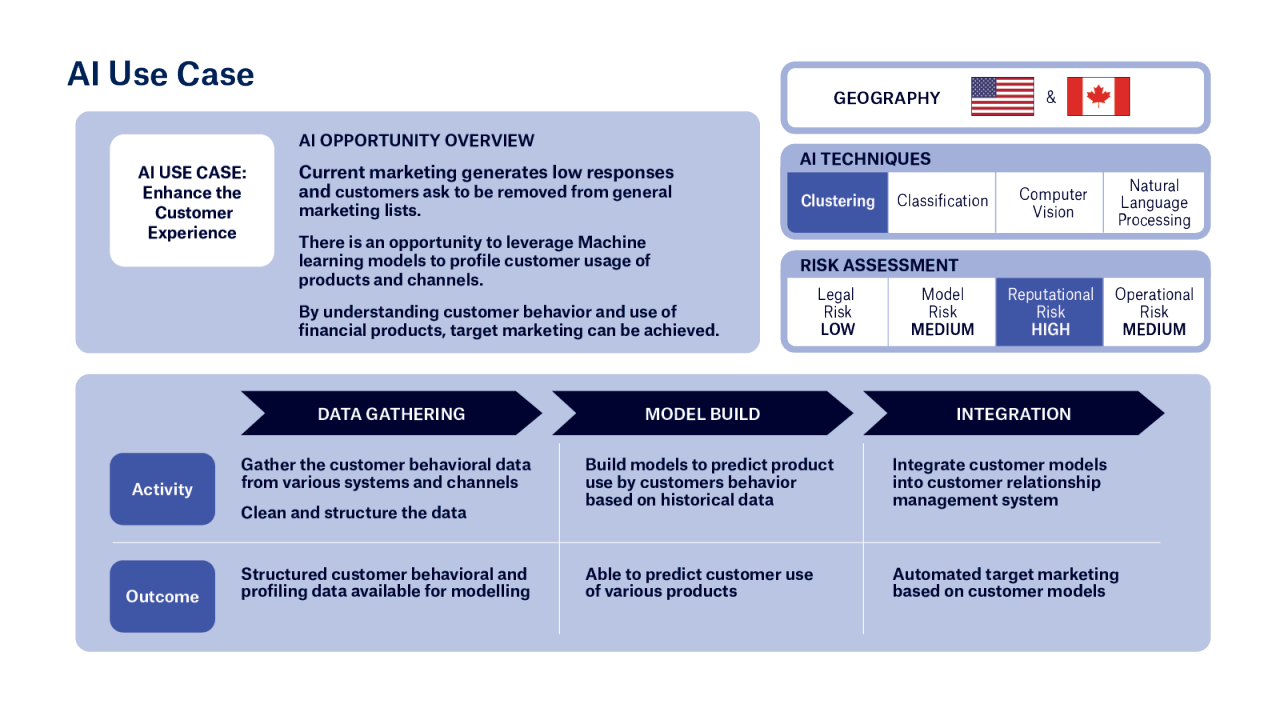
Takeaways
A successful AI strategy starts with establishing a clear vision and objectives. Clearly documented use cases are a must and will bring an AI strategy to life, creating the important link between AI and the benefits to the business.
Although each insurer’s AI strategy will be unique, the well-defined process described above will help every company build an effective AI strategy – one that helps to ensure business success.
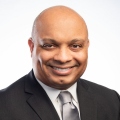